The Chatbox Market & 35 Essential Components Of Human-like Intelligence
Recommended soundtrack: Thunderstruck, AC/DC
This report has provided a comprehensive overview of the key functionalities and mathematical foundations of advanced chatbot systems. By examining 35 essential components of human-like intelligence, ranging from natural language understanding and generation to reasoning, learning, emotion, and self-awareness, we have highlighted the unique value and underlying mathematical concepts and techniques that enable their realization.
- Giddeon Gotnor, Founder
————————————-
- use your expense report to subscribe for the full report -
Company Note: Chatfuel
Company Report: Chatfuel
Major Market: Chatbot Market
Minor Market: Natural Language Processing (NLP) Engines
Address: 490 Post St STE 526, San Francisco, San Francisco
Introduction
Chatfuel, a pioneering force in the chatbot development market, has recently unveiled a suite of autonomous generative AI agents, marking a significant evolution from its traditional chatbot offerings. Founded in 2015 and headquartered in San Francisco, California, Chatfuel aims to simplify how businesses communicate with customers through conversational AI. As an official Meta partner, Chatfuel's no-code platform powers over a billion conversations each month for its 7 million+ business clients across Facebook Messenger, Instagram, and WhatsApp.
Product and Technology
Chatfuel's latest innovation, AI Sales Assistants, moves beyond simple chatbots to offer end-to-end sales process automation. These AI agents leverage advanced natural language processing and machine learning to handle complex customer interactions, from lead qualification and product recommendations to order processing and after-sales support. The platform's architecture revolves around visual bot building, NLP, integration management, and analytics, abstracting the underlying complexity into an intuitive interface accessible to non-technical users.
Chatfuel's AI agents are trained on the company's analysis of various sales methodologies, with skills distributed across specialized agents to ensure optimal performance in their respective roles. The agents tightly integrate with CRM systems and can be deployed in as little as 10 minutes, enabling rapid scaling for e-commerce SMBs.
Market Position and Differentiation
Chatfuel's AI Sales Assistants position the company at the forefront of the conversational AI market for e-commerce. By democratizing access to sophisticated AI automation, Chatfuel empowers businesses of all sizes to leverage the technology for growth without requiring deep technical expertise. Early results from beta customers showcase the transformative potential, with one SMB reducing hiring costs by 300% and another achieving sales conversions without human intervention.
Chatfuel's user-friendly platform, robust integration capabilities, and focus on popular messaging channels give it a strong competitive advantage in the rapidly growing conversational AI space. As the company expands its AI agents' capabilities to cover more business functions, it is poised to become an even more comprehensive solution for automation-driven growth.
Market Opportunity
The global chatbot market presents a significant growth opportunity, with projections indicating a substantial increase in market size over the coming years. According to multiple research firms, the chatbot market is expected to grow from USD 4.9 billion to an impressive USD 32 billion during the forecast period. This represents a remarkable Compound Annual Growth Rate (CAGR) of 31%, highlighting the rapid adoption and increasing demand for chatbot solutions across various industries.
Within the e-commerce industry specifically, chatbots are becoming increasingly crucial for enhancing customer engagement, streamlining sales processes, and providing personalized recommendations. As online shopping continues to grow, businesses are recognizing the need for automated solutions to handle high volumes of customer interactions efficiently.
The chatbot market's expansion is part of a broader trend in the conversational AI space. The global conversational AI market is expected to reach $32.62 billion by 2030, demonstrating the immense potential and demand for AI-driven communication solutions.
Chatfuel's strong focus on providing chatbot and AI agent solutions tailored for e-commerce positions the company strategically to capitalize on this sector's rapid adoption of conversational AI. As more e-commerce businesses seek to automate their sales and customer support functions, Chatfuel's offerings are well-aligned with the market's evolving needs.
Technical Architecture
While Chatfuel doesn't disclose all architectural details, key confirmed elements include its visual bot builder, AI-powered NLP, DialogFlow integration, and APIs for connecting with Facebook, Instagram, WhatsApp, and other third-party platforms. It delivers these capabilities through a SaaS model, likely leveraging cloud infrastructure, granular access controls, and advanced monitoring. The use of multiple large language models, each optimized for specific tasks, enables Chatfuel's AI agents to deliver peak performance.
Latest Updates
Chatfuel has launched four out-of-the-box AI agents, each tailored for specific use cases: a first-line support agent for handling FAQs, an SDR agent for lead qualification, an e-commerce sales associate for assisting with the order process, and a pre-sales manager for scheduling meetings. These agents can automate entire roles from start to finish with minimal human intervention, serving as a full-fledged AI sales department.
Chatfuel AI agents work by creating and prioritizing tasks based on the given goal, gathering information from the internet and other AI models, and iteratively refining their approach based on feedback until the goal is achieved. Businesses can easily deploy these agents in a matter of minutes by providing context about their operations and customers.
Bottom Line
Chatfuel's introduction of autonomous AI agents marks a significant leap in its mission to democratize conversational AI for business growth. By enabling the automation of entire sales roles through user-friendly, no-code tools, Chatfuel is empowering e-commerce businesses of all sizes to scale rapidly and cost-effectively.
As Chatfuel continues to enhance its AI agents' capabilities and expand into new business functions, it is well-positioned to lead the charge in the era of AI-driven automation. The company's strong track record, extensive meta integration, and commitment to continuous innovation make it a top pick for businesses seeking to harness the transformative potential of conversational AI.
With the chatbot and broader conversational AI markets poised for explosive growth, Chatfuel's pioneering AI agent technology places it at the vanguard of this revolution. As more businesses recognize the imperative of automation in the digital age, Chatfuel is set to play an increasingly pivotal role in shaping the future of business-customer interaction.
———————-
Competitors
Chatfuel operates in a dynamic and rapidly growing market, with numerous players offering chatbot development platforms and AI-powered conversational solutions. Some of Chatfuel's key competitors include:
1. MobileMonkey: A popular chatbot platform focusing on Facebook Messenger and Instagram integration, MobileMonkey offers a user-friendly interface for creating and deploying chatbots.
2. ManyChat: Another major player in the chatbot market, ManyChat enables businesses to build chatbots for Facebook Messenger, Instagram, and SMS. It provides a visual drag-and-drop interface and a range of templates and integrations.
3. Dialogflow: Developed by Google, Dialogflow is a natural language understanding platform that allows developers to create conversational interfaces for websites, mobile apps, and messaging platforms. While more developer-oriented than Chatfuel, it offers powerful AI capabilities.
4. Botkit: Botkit is an open-source framework for building chatbots and conversational apps. It supports multiple platforms, including Slack, Facebook Messenger, and Microsoft Teams, and provides a flexible development environment for more technical users.
5. Intercom: Intercom offers a customer messaging platform that includes chatbot capabilities. It focuses on customer engagement, conversion, and support, with features like targeted messaging, lead generation, and help desk automation.
——————————————————————-
Full reports are available with subscription.
Vendors wishing to see their full report or modify their reports can contact Giddeon Gotnor, Founder IBIDG.
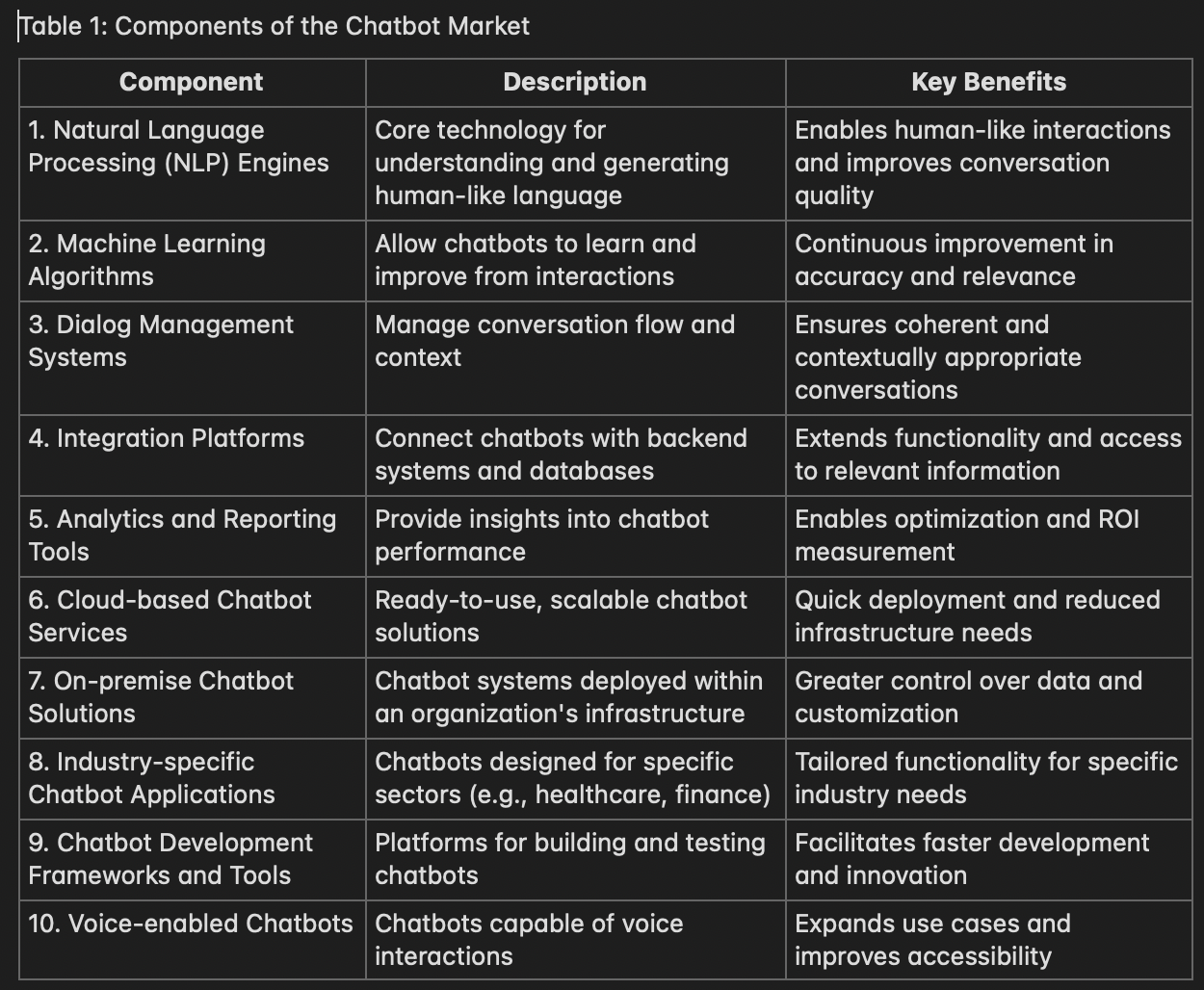
Components Of The Chatbox Market
The chatbot market is a rapidly evolving ecosystem that encompasses a wide range of technologies and applications. At its core are Natural Language Processing (NLP) engines, which form the foundation of chatbots by enabling human-like language understanding and response generation. These engines work in tandem with Machine Learning algorithms, providing adaptability and continuous improvement, allowing chatbots to become more accurate and relevant over time as they interact with users.
To ensure coherent and contextually appropriate conversations, Dialog Management Systems play a crucial role in enhancing user experience. These systems work alongside Integration Platforms, which extend chatbot capabilities by connecting them with various data sources and backend systems, increasing their utility and effectiveness across different business processes.
The performance and impact of chatbots are measured and optimized through Analytics and Reporting Tools. These tools offer valuable insights for businesses to refine their chatbot strategies and measure return on investment, driving continuous improvement in chatbot deployments.
The market offers flexibility in deployment options to cater to diverse business needs. Cloud-based Chatbot Services provide scalability and ease of deployment, making chatbot technology accessible to businesses of all sizes. For organizations with specific security or customization requirements, On-premise Chatbot Solutions offer greater control over data and functionality.
To address the unique needs of different sectors, Industry-specific Chatbot Applications deliver tailored solutions, improving efficiency and user engagement in specialized contexts such as healthcare, finance, and customer service. This specialization is further supported by Chatbot Development Frameworks and Tools, which democratize chatbot creation, enabling faster development and innovation even for organizations without extensive AI expertise.
As the market expands, Voice-enabled Chatbots are gaining prominence, extending the reach of conversational AI to new interfaces and use cases. This advancement enhances accessibility and user convenience, opening up new possibilities for hands-free interactions in various environments.
The chatbot market's growth is evidenced by impressive metrics seen across industries, including significant reductions in response times, improvements in customer satisfaction, increases in lead generation, and substantial cost savings. For instance, e-commerce companies have reported up to 35% reduction in response times and 25% increase in customer satisfaction scores. Financial services firms have seen 50% increases in qualified leads, while healthcare providers have achieved 60% reductions in appointment scheduling times.
As these technologies continue to mature and integrate, we're witnessing a transformation in how businesses interact with customers and manage internal operations. The synergy between NLP, machine learning, and specialized applications is driving improvements in natural language understanding, contextual awareness, and overall chatbot performance. This progress is leading to wider adoption across industries and use cases, from customer service and sales to internal operations and specialized industry applications.
Looking ahead, the chatbot market is poised for further growth and innovation. As businesses increasingly recognize the value of conversational AI in enhancing customer experiences and streamlining operations, we can expect to see even more sophisticated applications emerge. The ongoing advancements in each component of the chatbot ecosystem will likely lead to more natural, efficient, and valuable interactions, further solidifying chatbots as a crucial component of modern business strategies and customer engagement initiatives.
40 Chatbox Vendors
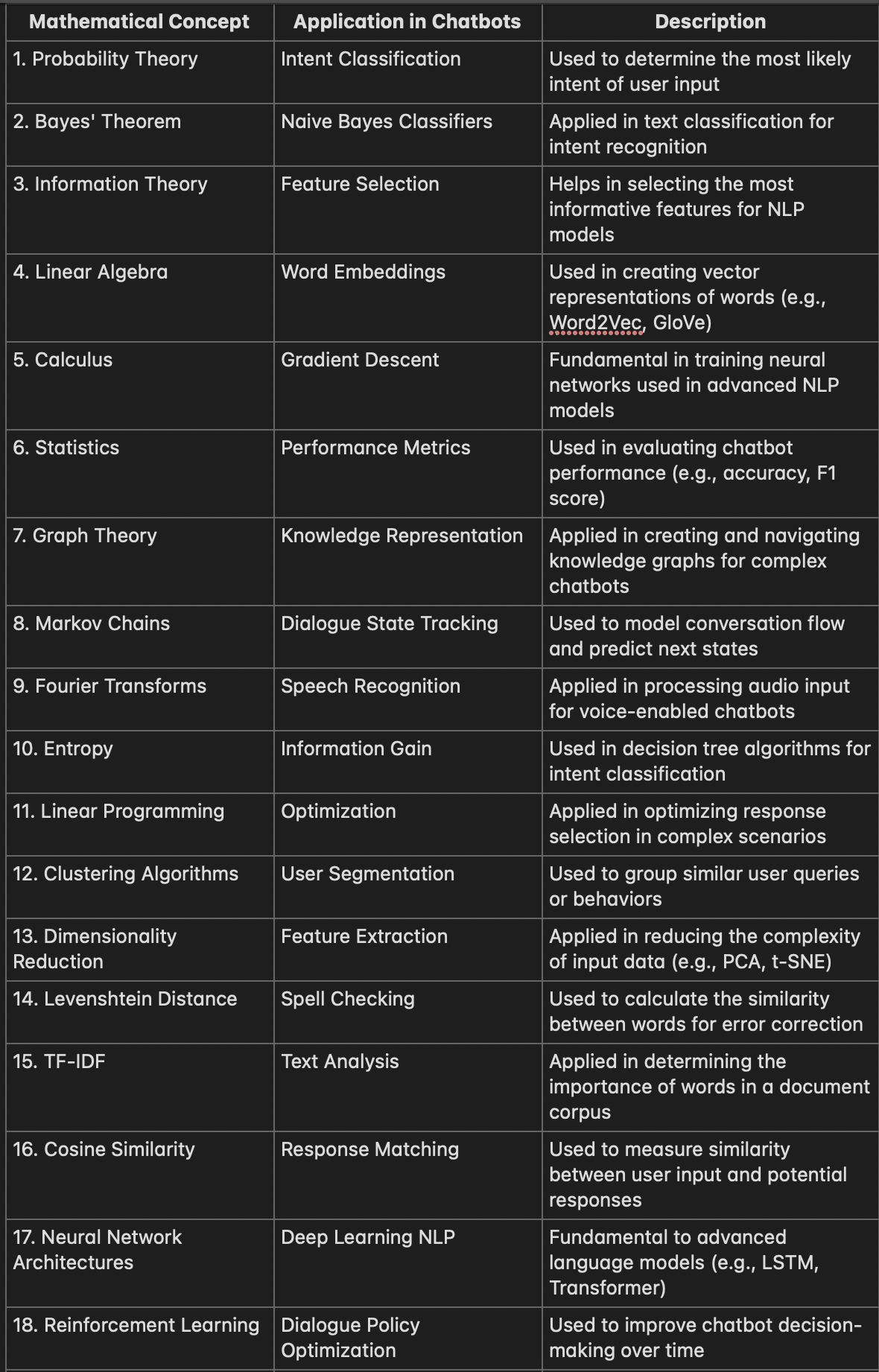
Research Note: Chatbox Mathematics
Report: Mathematical Foundations of Chatbot Technology
Probability Theory (1654)
Probability theory, pioneered by Blaise Pascal and Pierre de Fermat, is the mathematical study of random phenomena. It provides a framework for quantifying uncertainty and making predictions based on available information.
In the context of chatbots, probability theory is fundamental to intent classification and response selection. It allows chatbots to determine the most likely meaning of user input and choose the most appropriate response. The unique value of probability theory in chatbots is its ability to handle ambiguity and uncertainty in natural language, improving the accuracy and relevance of chatbot interactions.
Probability theory was initially developed to analyze games of chance, but its applications have expanded dramatically. In chatbots, its value is measured through improved accuracy rates in intent classification and user satisfaction scores.
Bayes' Theorem (1763)
Bayes' Theorem, formulated by Thomas Bayes, is a fundamental principle in probability theory that describes how to update the probability of a hypothesis given new evidence.
In chatbots, Bayes' Theorem is crucial for implementing Naive Bayes classifiers, which are used for text classification and intent recognition. It allows chatbots to learn from user interactions and improve their understanding over time. The unique value of Bayes' Theorem in chatbots is its ability to handle classification problems with limited training data efficiently.
Bayes' Theorem was developed as a solution to inverse probability problems. Its use in chatbots stems from its effectiveness in text classification tasks. The value it provides is measured through improved accuracy in intent recognition and the chatbot's ability to learn from new interactions.
Information Theory (1948)
Information theory, developed by Claude Shannon, is a mathematical theory that quantifies information and studies the transmission, processing, and storage of information.
In chatbot development, information theory is crucial for feature selection in Natural Language Processing (NLP) models. It helps determine which features (words or phrases) are most informative for understanding user intent. The unique value of information theory in chatbots is its ability to optimize the use of available data, improving efficiency and accuracy.
Information theory was originally developed for communication systems but has found wide applications in various fields. In chatbots, its value is measured through improved model efficiency and reduced computational requirements.
Linear Algebra (1950s-present)
Linear algebra, while having ancient roots, became crucial in modern computing in the mid-20th century. It deals with vector spaces and linear mappings between these spaces.
In chatbots, linear algebra is fundamental to creating word embeddings, which are vector representations of words. These embeddings capture semantic relationships between words, allowing chatbots to understand context and meaning better. The unique value of linear algebra in chatbots is its ability to represent complex language concepts in a form that machines can process efficiently.
Linear algebra's modern applications in computing were developed to solve systems of linear equations efficiently. Its use in chatbots is driven by the need to represent language mathematically. The value is measured through improved natural language understanding and processing speed.
Calculus (17th century, applied to ML in 1980s)
Calculus, developed independently by Newton and Leibniz in the 17th century, is the mathematical study of continuous change. Its application to machine learning became prominent in the 1980s.
In chatbot development, calculus is crucial for training neural networks through techniques like gradient descent. It allows the optimization of complex models with millions of parameters. The unique value of calculus in chatbots is its ability to fine-tune models for better performance continually.
While calculus was initially developed for physics problems, its application in machine learning arose from the need to optimize complex models. In chatbots, its value is measured through improved model performance and faster training times.
Statistics (18th-19th century)
Statistics, which saw significant development in the 18th and 19th centuries, is the discipline that concerns the collection, organization, analysis, interpretation, and presentation of data.
In chatbots, statistics is crucial for evaluating performance through metrics like accuracy, precision, recall, and F1 score. It also plays a role in analyzing user interaction data to improve the chatbot's functionality. The unique value of statistics in chatbots is its ability to provide quantitative insights into the chatbot's performance and user behavior.
Statistics was developed to handle and interpret large amounts of data. Its use in chatbots is driven by the need to measure and improve performance objectively. The value is measured through enhanced ability to quantify and improve chatbot effectiveness.
Graph Theory (1736)
Graph theory, initiated by Leonhard Euler in 1736, is the study of graphs, which are mathematical structures used to model pairwise relations between objects.
In advanced chatbots, graph theory is applied in creating and navigating knowledge graphs. These graphs represent complex relationships between entities, allowing chatbots to understand and reason about complex topics. The unique value of graph theory in chatbots is its ability to model and traverse complex information structures efficiently.
Graph theory was initially developed to solve abstract mathematical problems. Its application in chatbots arose from the need to represent and navigate complex knowledge structures. The value is measured through improved ability to handle complex queries and provide context-aware responses.
Markov Chains (1906)
Markov chains, introduced by Andrey Markov, are mathematical systems that transition from one state to another according to certain probabilistic rules.
In chatbots, Markov chains are used for dialogue state tracking, helping to model the flow of conversation and predict likely next states. This allows chatbots to maintain context and coherence in conversations. The unique value of Markov chains in chatbots is their ability to model sequential data efficiently.
Markov chains were developed to analyze sequences of random events. Their use in chatbots stems from the need to model and predict conversation flow. The value is measured through improved coherence and context-awareness in chatbot conversations.
Fourier Transforms (1822)
Fourier transforms, developed by Joseph Fourier, are mathematical transformations used to convert signals between time (or spatial) domain and frequency domain representations.
In voice-enabled chatbots, Fourier transforms are crucial for speech recognition, helping to process and analyze audio input. They allow the conversion of sound waves into frequency spectra that can be analyzed by machine learning models. The unique value of Fourier transforms in chatbots is their ability to extract meaningful features from audio signals.
Fourier transforms were initially developed for heat transfer problems but found wide applications in signal processing. Their use in chatbots is driven by the need to process audio input effectively. The value is measured through improved accuracy in speech recognition for voice-enabled chatbots.
Entropy (1850s)
Entropy, a concept from thermodynamics adapted to information theory by Claude Shannon in the 1940s, measures the average amount of information contained in a message.
In chatbot development, entropy is used to calculate information gain, which is crucial in decision tree algorithms for intent classification. It helps determine which features are most informative for making classification decisions. The unique value of entropy in chatbots is its ability to quantify the informativeness of different features or decisions.
Entropy was originally a concept in thermodynamics but was adapted to information theory to quantify information. Its use in chatbots stems from the need to make optimal decisions in classification tasks. The value is measured through improved efficiency and accuracy in intent classification.
Linear Programming (1939)
Linear programming, developed by Leonid Kantorovich in 1939, is a method to achieve the best outcome in a mathematical model whose requirements are represented by linear relationships.
In complex chatbot systems, linear programming can be applied to optimize response selection, especially in scenarios with multiple constraints or objectives. It allows chatbots to make optimal decisions based on various factors. The unique value of linear programming in chatbots is its ability to find optimal solutions in complex decision-making scenarios.
Linear programming was developed to solve resource allocation problems in economics. Its application in chatbots arose from the need to make optimal decisions in complex scenarios. The value is measured through improved decision-making capability and efficiency in resource allocation within the chatbot system.
Clustering Algorithms (1950s)
Clustering algorithms, which saw significant development in the 1950s, are unsupervised learning techniques used to group similar data points.
In chatbot development, clustering algorithms are used for user segmentation, grouping similar user queries or behaviors. This allows chatbots to provide more personalized responses and improve user experience. The unique value of clustering in chatbots is its ability to discover patterns in user behavior without predefined categories.
Clustering algorithms were developed to find natural groupings in data. Their use in chatbots is driven by the need to understand and categorize user behavior and queries. The value is measured through improved personalization and user satisfaction.
Dimensionality Reduction (1901, PCA)
Dimensionality reduction techniques, with Principal Component Analysis (PCA) introduced in 1901, are methods for reducing the number of random variables under consideration.
In chatbots, dimensionality reduction is applied in feature extraction, reducing the complexity of input data while retaining important information. This is crucial for processing high-dimensional text data efficiently. The unique value of dimensionality reduction in chatbots is its ability to make complex language data more manageable and interpretable.
Dimensionality reduction was developed to handle high-dimensional data in various fields. Its use in chatbots stems from the need to process complex language data efficiently. The value is measured through improved processing speed and model interpretability.
Levenshtein Distance (1965)
Levenshtein distance, introduced by Vladimir Levenshtein in 1965, is a string metric for measuring the difference between two sequences.
In chatbots, Levenshtein distance is used for spell checking and error correction. It allows chatbots to understand user input even when it contains typos or spelling errors. The unique value of Levenshtein distance in chatbots is its ability to handle imperfect user input, improving the robustness of the chatbot.
Levenshtein distance was developed for information theory and computer science applications. Its use in chatbots is driven by the need to handle and correct errors in user input. The value is measured through improved input understanding and user experience.
TF-IDF (Term Frequency-Inverse Document Frequency) (1972)
TF-IDF, introduced in its current form by Karen Spärck Jones in 1972, is a numerical statistic that reflects the importance of a word in a document within a collection.
In chatbot development, TF-IDF is used for text analysis, helping to determine the importance of words in a corpus of documents or user queries. This aids in understanding user intent and generating relevant responses. The unique value of TF-IDF in chatbots is its ability to identify key terms and concepts in user input.
TF-IDF was developed for information retrieval and text mining. Its application in chatbots stems from the need to understand the relative importance of words in user queries. The value is measured through improved relevance of chatbot responses and better understanding of user intent.
Cosine Similarity (1950s)
Cosine similarity, while based on earlier concepts, became widely used in computing in the 1950s. It measures the cosine of the angle between two non-zero vectors in an inner product space.
In chatbots, cosine similarity is used for response matching,
measuring the similarity between user input and potential responses. This helps in selecting the most appropriate response from a set of possibilities. The unique value of cosine similarity in chatbots is its ability to compare text vectors efficiently, regardless of their magnitude.
Cosine similarity was developed for measuring document similarity in information retrieval. Its use in chatbots is driven by the need to match user queries with appropriate responses efficiently. The value is measured through improved response relevance and reduced response time.
Neural Network Architectures (1943, modern era 1980s-present)
Neural networks, while conceptualized in 1943, saw significant development in the 1980s and have evolved rapidly since then. They are computing systems inspired by biological neural networks.
In chatbots, advanced neural network architectures like LSTM (Long Short-Term Memory) and Transformers are fundamental to deep learning NLP models. They enable sophisticated language understanding and generation. The unique value of neural networks in chatbots is their ability to learn complex patterns in language data, leading to more human-like interactions.
Neural networks were developed to mimic human brain function. Their application in chatbots arose from the need for more sophisticated language processing capabilities. The value is measured through improved natural language understanding and generation, leading to more engaging and accurate chatbot interactions.
Reinforcement Learning (1950s, modern applications 1980s-present)
Reinforcement learning, while having roots in the 1950s, saw significant development from the 1980s onward. It's a type of machine learning where an agent learns to make decisions by taking actions in an environment to maximize a reward.
In chatbot development, reinforcement learning is used for dialogue policy optimization. It allows chatbots to learn optimal conversation strategies through trial and error, improving decision-making over time. The unique value of reinforcement learning in chatbots is its ability to adapt and improve conversational strategies based on user interactions.
Reinforcement learning was developed to solve sequential decision-making problems. Its application in chatbots stems from the need to optimize conversational strategies dynamically. The value is measured through improved long-term conversation quality and user satisfaction.
Fuzzy Logic (1965)
Fuzzy logic, introduced by Lotfi Zadeh in 1965, is a form of many-valued logic in which the truth values of variables may be any real number between 0 and 1.
In chatbots, fuzzy logic is applied in handling uncertainty and ambiguity in user inputs. It allows chatbots to make decisions based on partial or imprecise information, leading to more flexible and human-like reasoning. The unique value of fuzzy logic in chatbots is its ability to handle the inherent vagueness in natural language.
Fuzzy logic was developed to handle imprecise and qualitative concepts mathematically. Its use in chatbots is driven by the need to handle ambiguous or uncertain user inputs more effectively. The value is measured through improved handling of complex or unclear user queries.
Time Series Analysis (18th century, modern applications mid-20th century)
Time series analysis, while having roots in the 18th century, saw significant development in the mid-20th century. It involves methods for analyzing time series data to extract meaningful statistics and characteristics.
In advanced chatbot systems, time series analysis is used for predictive analytics, forecasting user behavior or query patterns over time. This allows chatbots to anticipate user needs and provide proactive assistance. The unique value of time series analysis in chatbots is its ability to identify trends and patterns in user interactions over time.
Time series analysis was developed to analyze data points collected over time. Its application in chatbots stems from the need to understand and predict user behavior patterns. The value is measured through improved proactive capabilities and personalization of chatbot interactions over time.
Strategic Planning Assumption: An Aircraft Carrier Will Arrive Over These Locations (Probability .69)
Estimated to contain more wealth than Fort Knox:
1) 13°22'26.75"S 87°52'2.37"E:
2) 13°22'29.39"S 87°51'18.71"E
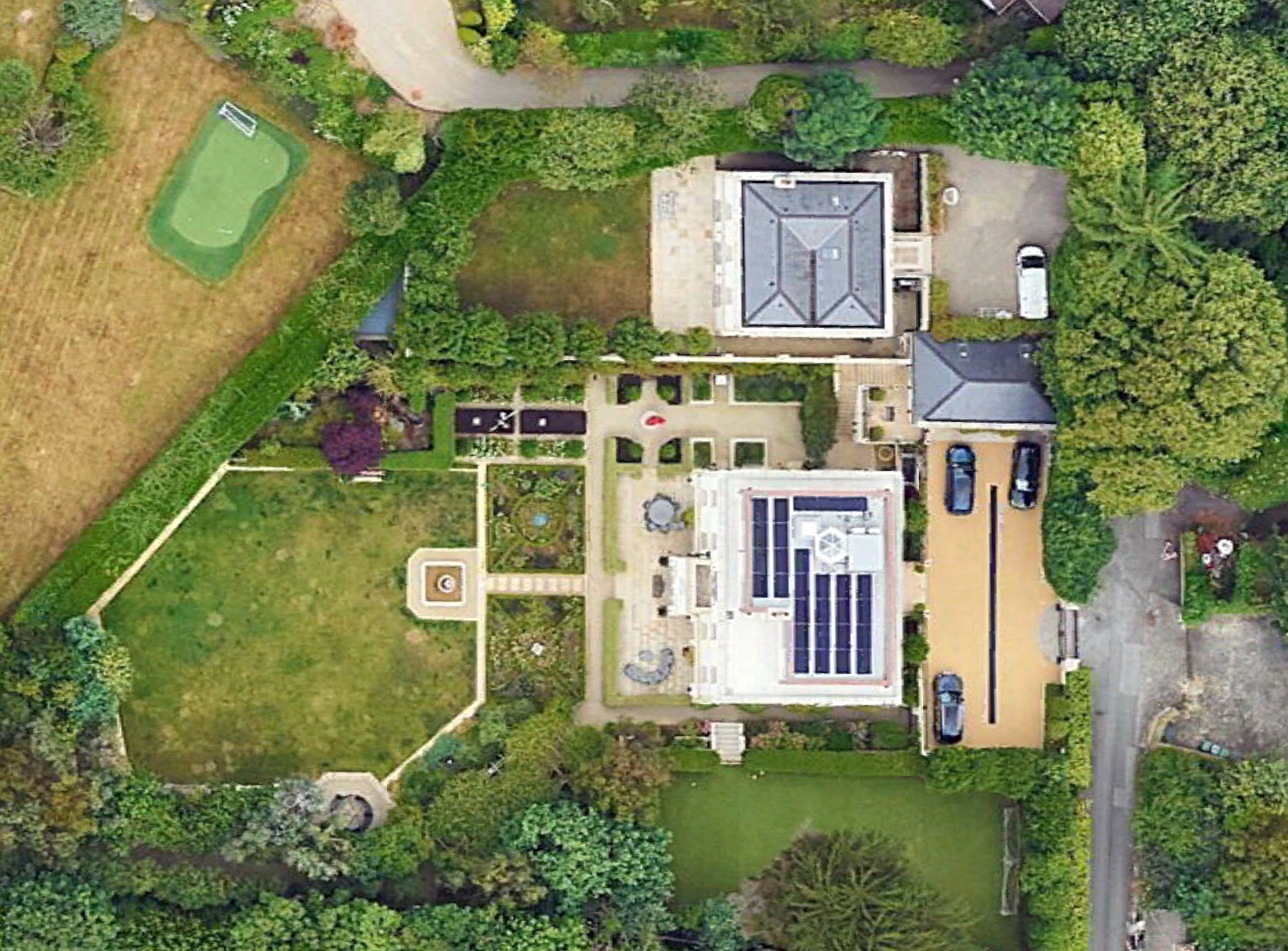
Research Report: The Evolution of Mathematics in Artificial Intelligence Introduction:
Recommended soundtrack: You’re Welcome, Dwayne Johnson
The Evolution of Mathematics in Artificial Intelligence
Introduction:
The development of artificial intelligence (AI) has been fundamentally shaped by advancements in mathematics. This report examines the evolutionary trajectory of mathematical concepts that have been crucial to AI, spanning from the 17th century to the present day. We will explore how each mathematical breakthrough has contributed to the field, its historical context, and its impact on AI capabilities.
Component Evolved: Reasoning under uncertainty (Probability Theory, 1654)
What is the component?
Probability theory provides a mathematical framework for quantifying and reasoning about uncertain events and outcomes.
Why did it evolve?
In the mid-17th century, gamblers and mathematicians sought to understand games of chance more rigorously. This led to the development of probability theory by Blaise Pascal and Pierre de Fermat.
What was used previous to its discovery?
Before probability theory, reasoning about uncertain events relied on intuition, superstition, and simple heuristics. There was no formal mathematical framework for quantifying the likelihood of events.
Net benefit and performance gain:
Probability theory laid the groundwork for statistical inference and decision-making under uncertainty. In AI, it enables systems to handle noisy data, make predictions, and quantify confidence in their outputs. Performance gains can be measured through improved accuracy in predictions and more robust decision-making in uncertain environments.
Component Evolved: Probabilistic inference (Bayes' Theorem, 1763)
What is the component?
Bayes' Theorem provides a method for updating probabilities based on new evidence, forming the basis for Bayesian inference.
Why did it evolve?
Thomas Bayes sought to solve the problem of inverse probability – determining causes from observed effects.
What was used previous to its discovery?
Prior to Bayes' Theorem, probabilistic reasoning was largely limited to direct probability calculations and did not have a formal method for incorporating new evidence.
Net benefit and performance gain:
Bayes' Theorem enables AI systems to learn from data and update their beliefs dynamically. This has led to more adaptive and accurate AI models, particularly in areas like spam filtering, medical diagnosis, and recommendation systems. Performance gains are evident in improved accuracy and the ability to handle complex, real-world scenarios with incomplete information.
Component Evolved: Sequential modeling (Markov Chains, 1906)
What is the component?
Markov Chains model systems that transition between states, where the probability of each transition depends only on the current state.
Why did it evolve?
Andrey Markov developed this concept to model sequences of dependent random variables, initially applied to analyzing sequences of letters in literature.
What was used previous to its discovery?
Before Markov Chains, most probabilistic models assumed independence between events, limiting their ability to capture sequential or time-dependent phenomena.
Net benefit and performance gain:
Markov Chains have enabled AI systems to model and predict sequential data more effectively. This has been crucial in natural language processing, speech recognition, and time series analysis. Performance gains can be measured through improved accuracy in tasks like text generation, speech synthesis, and predictive modeling of sequential processes.
Component Evolved: Quantification of information (Information Theory, 1948)
What is the component?
Information theory provides a mathematical framework for quantifying information content and the limits of data compression and transmission.
Why did it evolve?
Claude Shannon developed information theory to address fundamental limits of communication and data compression in the context of emerging telecommunications technologies.
What was used previous to its discovery?
Before information theory, there was no formal way to quantify information content or to determine the theoretical limits of data compression and error-free communication.
Net benefit and performance gain:
Information theory has been crucial in developing efficient data compression algorithms, error-correcting codes, and in understanding the limits of machine learning models. In AI, it has informed the development of more efficient neural network architectures and training algorithms. Performance gains can be measured through improved data compression ratios, more robust communication systems, and more efficient AI models.
Component Evolved: Neural network training (Backpropagation, 1986)
What is the component?
Backpropagation is an algorithm for efficiently training multi-layer neural networks by computing the gradient of the loss function with respect to the network's weights.
Why did it evolve?
There was a need for an efficient method to train multi-layer neural networks, as previous attempts were computationally expensive and often failed to converge.
What was used previous to its discovery?
Before backpropagation, neural networks were limited to single-layer architectures (perceptrons) or used less efficient training methods like random weight updates or layer-by-layer training.
Net benefit and performance gain:
Backpropagation enabled the training of deep neural networks, leading to significant breakthroughs in various AI tasks. Performance gains are evident in the dramatic improvements in accuracy and capability of neural networks across domains like computer vision, speech recognition, and natural language processing.
Component Evolved: Non-linear classification and regression (Support Vector Machines, 1992)
What is the component?
Support Vector Machines (SVMs) are a class of algorithms that find optimal hyperplanes for classification and regression tasks in high-dimensional spaces.
Why did it evolve?
Vladimir Vapnik and Alexey Chervonenkis developed SVMs to address limitations in existing classification methods, particularly for handling non-linearly separable data.
What was used previous to its discovery?
Prior to SVMs, classification tasks primarily relied on linear models or simple neural networks, which struggled with complex, non-linear decision boundaries.
Net benefit and performance gain:
SVMs introduced the concept of maximum margin classifiers and the kernel trick, enabling effective non-linear classification and regression. They have shown superior performance in many machine learning tasks, especially with small to medium-sized datasets. Gains can be measured through improved classification accuracy and better generalization to unseen data.
Component Evolved: Learning through interaction (Reinforcement Learning, formalized 1998)
What is the component?
Reinforcement Learning (RL) is a framework for learning optimal decision-making strategies through interaction with an environment.
Why did it evolve?
While the concepts of RL had been around earlier, Richard Sutton and Andrew Barto formalized the framework to address the challenge of learning optimal behaviors in complex, uncertain environments.
What was used previous to its discovery?
Before the formalization of RL, most machine learning focused on supervised or unsupervised learning paradigms, which were not well-suited for sequential decision-making problems.
Net benefit and performance gain:
RL has enabled AI systems to learn complex strategies in domains like game playing, robotics, and resource management. Performance gains are evident in achievements like defeating world champions in games like Go and poker, and in the development of more adaptive and autonomous robotic systems.
Component Evolved: Spatial feature learning (Convolutional Neural Networks, 1998)
What is the component?
Convolutional Neural Networks (CNNs) are a class of deep neural networks designed to process grid-like data, such as images.
Why did it evolve?
Yann LeCun and colleagues developed CNNs to address the limitations of fully connected networks in processing high-dimensional data with spatial structure.
What was used previous to its discovery?
Before CNNs, image processing tasks relied on hand-crafted feature extractors followed by traditional machine learning classifiers, or used fully connected neural networks which were computationally expensive and prone to overfitting.
Net benefit and performance gain:
CNNs have revolutionized computer vision tasks, achieving human-level or superhuman performance in image classification, object detection, and segmentation. Performance gains are measured through dramatic improvements in accuracy on benchmark datasets and the ability to process large-scale image and video data efficiently.
Component Evolved: Generative modeling (Generative Adversarial Networks, 2014)
What is the component?
Generative Adversarial Networks (GANs) are a framework for training generative models through an adversarial process.
Why did it evolve?
Ian Goodfellow introduced GANs to address limitations in existing generative models, particularly in generating realistic, high-quality samples.
What was used previous to its discovery?
Prior to GANs, generative modeling primarily relied on methods like variational autoencoders or restricted Boltzmann machines, which often produced blurry or unrealistic samples.
Net benefit and performance gain:
GANs have enabled the generation of highly realistic synthetic data across various domains, including images, text, and audio. Performance gains are evident in the quality and diversity of generated samples, as well as applications in data augmentation, style transfer, and domain adaptation.
Component Evolved: Attention-based sequence processing (Transformer Architecture, 2017)
What is the component?
The Transformer architecture is a neural network design that relies solely on attention mechanisms to process sequential data.
Why did it evolve?
Ashish Vaswani and colleagues developed the Transformer to address limitations of recurrent neural networks in processing long sequences and to enable more parallelization in training.
What was used previous to its discovery?
Before Transformers, sequence processing tasks primarily used recurrent neural networks (RNNs) like LSTMs or GRUs.
Net benefit and performance gain:
Transformers have achieved state-of-the-art performance in various natural language processing tasks, surpassing RNN-based models while requiring significantly less training time. Performance gains are measured through improved accuracy on benchmark tasks, reduced training time, and the ability to handle longer sequences more effectively.
Bottom Line
The evolution of mathematics in AI reflects a journey from foundational concepts of uncertainty and information to sophisticated algorithms for learning and generalization. Each advancement has expanded the capabilities of AI systems, enabling them to tackle increasingly complex problems across diverse domains. As AI continues to evolve, it is likely that further mathematical innovations will play a crucial role in overcoming current limitations and pushing the boundaries of artificial intelligence.
Artificial Intelligence Technology Predictions 2025-2034
“IBIDG’s enterprise artificial intelligence predictions are within the section. A thin man’s slice of heaven.”
-Giddeon Gotnor
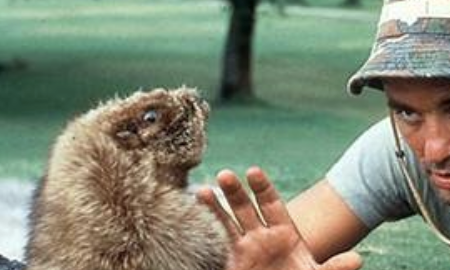
Caddyshack
Recommended soundtrack: I am al’ right, Kennie, Loggins
————————————————————————-
Caddyshack (1980, 30 Santa Bella Road) Report
Overview:
Caddyshack is a 1980 American sports comedy film directed by Harold Ramis. It was his directorial debut and became a cult classic, described by ESPN as "perhaps the funniest sports movie ever made."
Main Characters:
Danny Noonan (Michael O'Keefe): A high school student working as a caddie at Bushwood Country Club, hoping to earn a scholarship for college.
Ty Webb (Chevy Chase): The free-spirited son of one of the club's co-founders and a talented golfer.
Al Czervik (Rodney Dangerfield): A loud, eccentric nouveau riche real estate developer who antagonizes the club's establishment.
Judge Elihu Smails (Ted Knight): An arrogant co-founder and director of the club who oversees the caddie scholarship program (Juedge’s family goes to IVY League for services).
Carl Spackler (Bill Murray): The mentally unstable greenskeeper tasked with eliminating a troublesome gopher.
Plot Summary:
The film revolves around the interactions and conflicts at the exclusive Bushwood Country Club. Danny Noonan, a caddie, tries to win the favor of Judge Smails to secure a college scholarship. Meanwhile, the arrival of the boisterous Al Czervik disrupts the club's stuffy atmosphere, leading to tension with Smails.
A subplot involves Carl Spackler's increasingly extreme attempts to get rid of a persistent gopher damaging the golf course.
The story culminates in a high-stakes golf match between Smails and Czervik's teams. When Czervik feigns injury, Danny steps in as his replacement. The match comes down to Danny's final putt, with his college future hanging in the balance. As Danny's ball teeters on the edge of the hole, Carl's latest gopher-killing explosion causes it to drop in, securing victory for Danny's team.
The film ends with the unharmed gopher dancing to Kenny Loggins' "I'm Alright," while the human characters deal with the aftermath of the match and Carl's destructive antics.
Caddyshack blends slapstick comedy, social satire, and sports movie tropes to create a unique and enduring comedy classic.
Gaza’s Ghost Ship
Recommended soundtrack: I Mean It, G- Eazy
Recommended soundtrack: Jail Break, AC/DC
1) 31°25'55.44"N 34°20'43.69"E
2) 33°46'54.89"N 118°21'31.73"W
”The Zodiac boarded an IVY League “ghost ship” from Gaza.” - Giddeon Gotnor, Founder
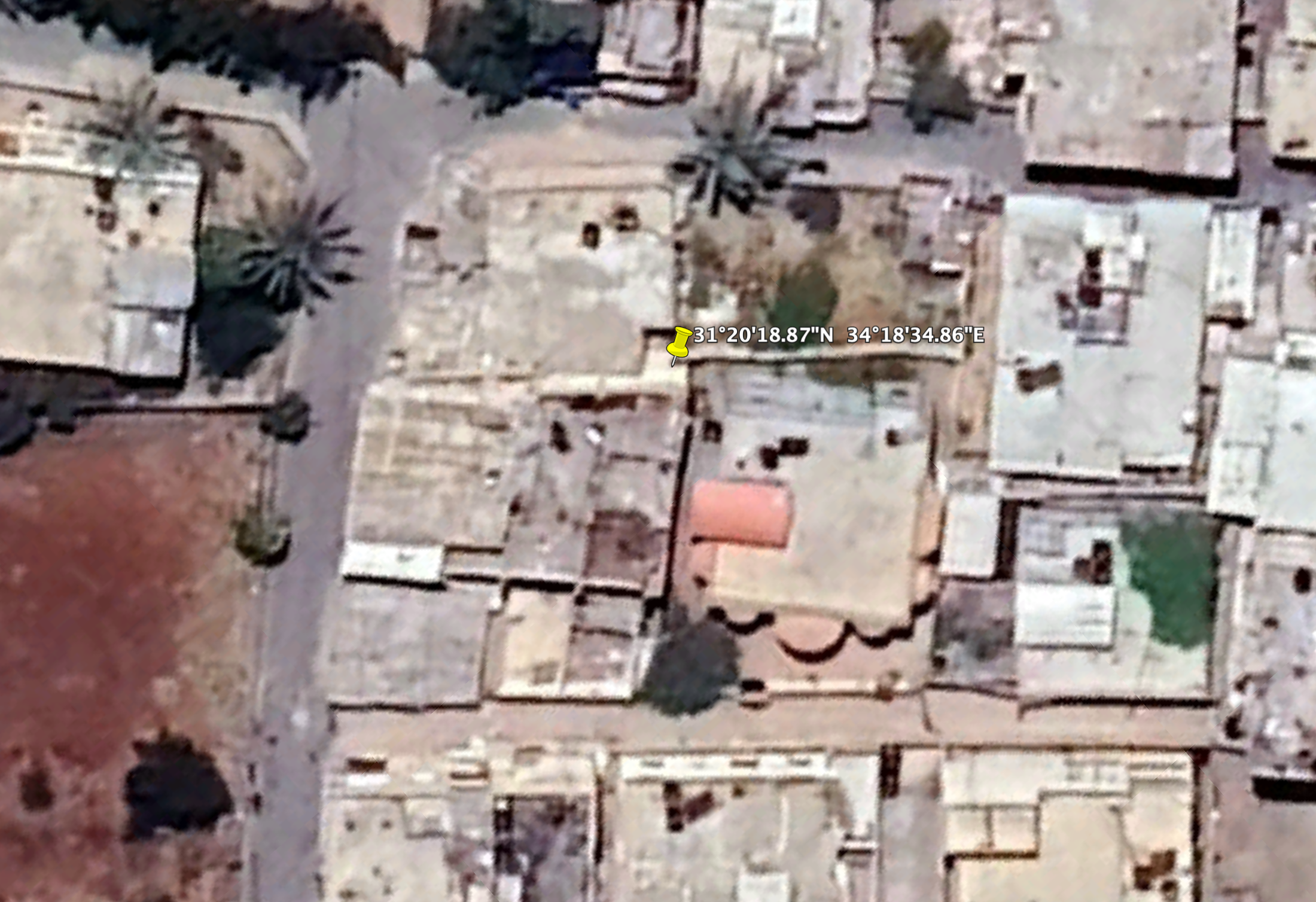
The Letter G
G: 31°20'18.87"N 34°18'34.86"E
The Evolution of the Machine Learning Industry
Recommended soundtrack: Cross Road Blues, Robert Johnson
Executive Summary
The Evolution of the Machine Learning Industry
The machine learning industry has undergone a remarkable evolution since its early beginnings in the mid-20th century. From the introduction of artificial neural networks and the concept of machines that could learn, to the development of advanced algorithms and the emergence of deep learning, machine learning has become an integral part of modern technology.
Key milestones in the evolution of machine learning include:
Early foundations and classical algorithms (1940s-1970s)
Introduction of artificial neural networks, concept of artificial intelligence, and development of optimization techniques.
The rise of statistical learning (1980s-1990s)
Emergence of reinforcement learning, convolutional neural networks (CNNs), recurrent neural networks (RNNs), ensemble methods, and transfer learning.
The deep learning revolution (2000s-2010s)
Development of hierarchical feature learning, graph neural networks, autoencoders, word embeddings, generative adversarial networks (GANs), attention mechanisms, and transformer models.
Recent innovations and future directions (late 2010s-present)
Focus on automated machine learning (AutoML), federated learning, self-supervised learning, neuro-symbolic AI, and quantum machine learning.
The machine learning industry is poised for continued growth and innovation, with the potential to transform various aspects of our lives. However, it is crucial to address the ethical and societal implications of these powerful technologies, ensuring that they are developed and deployed responsibly.
The Machine Learning Industry must focus on:
Improving interpretability and transparency of machine learning models.
Enhancing efficiency and scalability of machine learning systems.
Making machine learning more accessible to a wider range of users and applications.
Addressing ethical considerations and societal impacts of machine learning technologies.
Ensuring responsible development and deployment of machine learning solutions.
By prioritizing these key areas, the machine learning industry can continue to push the boundaries of what is possible and create value for society as a whole.

Market Note: Machine Learning
Recommended soundtrack: Voodoo Child, Jimmy Hendrix
Supervised Learning Algorithms
Supervised learning algorithms learn from labeled data, where both input features and target variables are provided. These algorithms aim to learn a function that maps inputs to outputs, allowing them to make predictions on new, unseen data.
Linear Regression
A method for modeling the linear relationship between a dependent variable and one or more independent variables. It's used for predicting continuous values and understanding the impact of features on the target variable.
Logistic Regression
Despite its name, this is a classification algorithm used for predicting binary outcomes. It estimates the probability of an instance belonging to a particular class.
Decision Trees
Tree-like models that make decisions based on asking a series of questions about the features. They're interpretable and can handle both classification and regression tasks.
Random Forests
An ensemble learning method that constructs multiple decision trees and merges them to get a more accurate and stable prediction. It's robust against overfitting and can handle high-dimensional data.
Support Vector Machines (SVM)
Algorithms that find the hyperplane that best separates classes in high-dimensional space. They're effective for both linear and non-linear classification tasks.
Naive Bayes
A probabilistic classifier based on applying Bayes' theorem with strong independence assumptions between features. It's particularly useful for text classification and spam filtering.
K-Nearest Neighbors (KNN)
A simple, instance-based learning algorithm that classifies new data points based on the majority class of their k nearest neighbors in the feature space.
Neural Networks
Inspired by biological neural networks, these are a series of algorithms that aim to recognize underlying relationships in a set of data through a process that mimics how the human brain operates.
Unsupervised Learning Algorithms
Unsupervised learning algorithms work with unlabeled data, trying to find hidden patterns or intrinsic structures. These are often used for clustering, dimensionality reduction, and anomaly detection.
K-Means Clustering
A method that aims to partition n observations into k clusters in which each observation belongs to the cluster with the nearest mean (cluster centroid).
Hierarchical Clustering
An algorithm that builds nested clusters by merging or splitting them successively. This creates a cluster hierarchy or a tree structure to represent the data.
Principal Component Analysis (PCA)
A technique used to emphasize variation and bring out strong patterns in a dataset. It's often used to reduce the dimensionality of datasets.
Independent Component Analysis (ICA)
A statistical method for separating a multivariate signal into additive subcomponents, assuming the mutual statistical independence of the source signals.
Apriori Algorithm
An algorithm for frequent item set mining and association rule learning over relational databases. It proceeds by identifying frequent individual items in the database.
Gaussian Mixture Models
A probabilistic model that assumes all the data points are generated from a mixture of a finite number of Gaussian distributions with unknown parameters.
Reinforcement Learning Algorithms
Reinforcement learning is about taking suitable action to maximize reward in a particular situation. It's employed in various fields, including game theory, control theory, operations research, and information theory.
Q-Learning
A model-free reinforcement learning algorithm to learn the value of an action in a particular state. It does not require a model of the environment and can handle problems with stochastic transitions and rewards.
SARSA (State-Action-Reward-State-Action)
An algorithm for learning a Markov decision process policy. This algorithm is an on-policy temporal difference (TD) control algorithm.
Deep Q Network (DQN)
An approach that combines Q-learning with artificial neural networks. DQNs can learn successful policies directly from high-dimensional sensory inputs.
Policy Gradient Methods
These methods learn a parameterized policy that can select actions without consulting a value function. A value function may still be used to learn the policy parameter, but is not required for action selection.
Deep Learning Algorithms
Deep learning is part of a broader family of machine learning methods based on artificial neural networks. It allows computational models composed of multiple processing layers to learn representations of data with multiple levels of abstraction.
Convolutional Neural Networks (CNN)
Primarily used for processing grid-like data such as images. CNNs use convolution in place of general matrix multiplication in at least one of their layers.
Recurrent Neural Networks (RNN)
Designed for processing sequential data. RNNs use internal state (memory) to process sequences of inputs, making them applicable to tasks such as speech recognition and language modeling.
Long Short-Term Memory Networks (LSTM)
A special kind of RNN capable of learning long-term dependencies. LSTMs are explicitly designed to avoid the long-term dependency problem.
Generative Adversarial Networks (GAN)
A class of machine learning frameworks. In a GAN, two neural networks contest with each other in a game. Given a training set, this technique learns to generate new data with the same statistics as the training set.
Transformer Models
Models that use self-attention mechanisms to process sequential data. They have become the foundation for many state-of-the-art models in natural language processing.
Ensemble Methods
Ensemble methods use multiple learning algorithms to obtain better predictive performance than could be obtained from any of the constituent learning algorithms alone.
Bagging
A method involving training multiple models on random subsets of the training data and then aggregating their predictions. Random Forests are a popular example of bagging.
Boosting
A family of algorithms that convert weak learners to strong ones. Popular boosting algorithms include AdaBoost and Gradient Boosting.
Stacking
An ensemble learning technique that uses predictions from multiple models (often of different types) to build a new model.
Dimensionality Reduction
These techniques are used to reduce the number of features in a dataset while retaining as much information as possible.
t-SNE
A technique for dimensionality reduction that is particularly well suited for the visualization of high-dimensional datasets.
UMAP
A dimension reduction technique that can be used for visualization similarly to t-SNE, but also for general non-linear dimension reduction.
Anomaly Detection
Anomaly detection is the identification of rare items, events or observations which raise suspicions by differing significantly from the majority of the data.
Isolation Forest
An unsupervised learning algorithm for detecting anomalies. It isolates anomalies instead of profiling normal points.
One-Class SVM
A type of support vector machine that learns a decision boundary to classify new data as similar or different to the training set.
Optimization Algorithms
These are algorithms used to find the best solution from all feasible solutions, particularly in training machine learning models.
Gradient Descent
An iterative optimization algorithm for finding the minimum of a function. It takes steps proportional to the negative of the gradient of the function at the current point.
Stochastic Gradient Descent (SGD)
A stochastic approximation of the gradient descent optimization method for minimizing an objective function that is written as a sum of differentiable functions.
Adam Optimizer
An algorithm for first-order gradient-based optimization of stochastic objective functions, based on adaptive estimates of lower-order moments.
Feature Selection
Feature selection methods are used to identify the most relevant features in a dataset, which can improve model performance and interpretability.
Lasso Regression
A regression analysis method that performs both variable selection and regularization to enhance the prediction accuracy and interpretability of the statistical model it produces.
Ridge Regression
A method of estimating the coefficients of multiple-regression models in scenarios where independent variables are highly correlated.
Elastic Net
A regularized regression method that linearly combines the L1 and L2 penalties of the lasso and ridge methods.
Time Series Analysis
Time series analysis comprises methods for analyzing time series data to extract meaningful statistics and other characteristics of the data.
ARIMA
A class of models that captures a suite of different standard temporal structures in time series data.
Prophet
A procedure for forecasting time series data based on an additive model where non-linear trends are fit with yearly, weekly, and daily seasonality, plus holiday effects.
Bottom Line
Machine learning encompasses a vast array of algorithms and techniques, each designed to tackle specific types of problems and data structures. From supervised learning methods that work with labeled data to unsupervised approaches that uncover hidden patterns, and from reinforcement learning that interacts with environments to deep learning that processes complex data structures, the field offers a rich toolkit for data scientists and researchers. Ensemble methods, dimensionality reduction, and feature selection techniques further enhance the capabilities of these core algorithms. As the field continues to evolve, new methods and refinements of existing techniques emerge, pushing the boundaries of what's possible in artificial intelligence and data analysis. The choice of algorithm depends on the specific problem, data characteristics, and desired outcomes, making a broad understanding of these methods crucial for effective application of machine learning.
——
( use your expense report for a subscription )
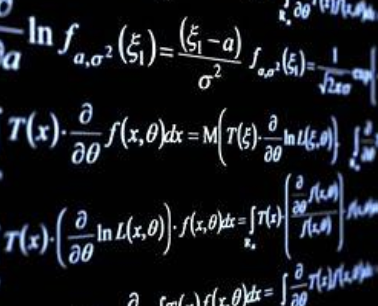
Mathematical Concepts and Techniques in AI
Recommeded soundtrack: Foolz Waltz
—————————————————————-
36 Mathematical Processes Are Reviewed
—————————————————————-
Executive Summary
Mathematical Clusters and Industry Applications
To better understand the role of mathematics in the AI market, we can cluster the mathematical concepts and techniques based on their relevance to specific industries and applications. These clusters highlight how different branches of mathematics contribute to solving real-world problems across various domains.
Healthcare and Life Sciences Verticals
a) Probability Theory and Bayesian Inference:
Used in medical diagnosis, drug discovery, and clinical decision support systems.
b) Graph Theory and Network Analysis:
Applied in analyzing biological networks, predicting disease progression, and identifying drug targets.
c) Optimization and Machine Learning:
Utilized in personalizing treatment plans, optimizing resource allocation, and predicting patient outcomes.
Example: In cancer diagnosis, machine learning algorithms like support vector machines (SVM) can be trained on medical imaging data to classify tumors as malignant or benign. The SVM algorithm finds an optimal hyperplane that separates the data points into different classes based on their features, enabling accurate and early detection of cancer.
Finance and Banking Verticles
a) Time Series Analysis and Forecasting:
Used in stock market prediction, risk assessment, and fraud detection.
b) Stochastic Calculus and Differential Equations:
Applied in pricing financial derivatives, portfolio optimization, and quantitative trading strategies.
c) Graph Theory and Network Analysis:
Employed in analyzing financial networks, detecting money laundering, and assessing systemic risk.
Example: Fraud detection systems in banking often rely on anomaly detection techniques like clustering and outlier analysis. By representing transaction data as points in a high-dimensional space and applying algorithms like k-means or DBSCAN, unusual patterns or outliers can be identified, indicating potential fraudulent activities.
Manufacturing and Supply Chain Verticles
a) Optimization and Operations Research:
Used in production planning, inventory management, and logistics optimization.
b) Time Series Analysis and Forecasting:
Applied in demand forecasting, predictive maintenance, and quality control.
c) Simulation and Stochastic Modeling:
Employed in analyzing supply chain risks, optimizing warehouse operations, and evaluating production scenarios.
Example: In predictive maintenance, machine learning algorithms like long short-term memory (LSTM) networks can be trained on sensor data from manufacturing equipment to predict potential failures. LSTM networks can capture long-term dependencies in the data, enabling accurate prediction of remaining useful life and optimizing maintenance schedules.
Marketing and Retail Vericals
a) Natural Language Processing and Text Analytics:
Used in sentiment analysis, customer feedback analysis, and content recommendation.
b) Time Series Analysis and Forecasting:
Applied in sales forecasting, demand prediction, and customer churn analysis.
c) Graph Theory and Network Analysis:
Employed in analyzing customer social networks, identifying influencers, and optimizing product recommendations.
Example: Recommender systems in e-commerce often use matrix factorization techniques like singular value decomposition (SVD) to predict user preferences. By decomposing the user-item rating matrix into lower-dimensional representations, the system can uncover latent factors that capture user and item characteristics, enabling personalized product recommendations.
( use your expense report to pay for subscription )

Research Report: The Benefits of 6G Technology
Recommended soundtrack: They say I’m different, Betty Davis
Introduction:
This report outlines the tangible expected benefits of 6G technology, organized by key components. Each section highlights specific improvements and their potential impact on various industries and applications.
1. Frequency Bands (Sub-THz and THz) Benefits:
* Enables ultra-high-definition holographic communications
* Supports massive machine-type communications for advanced IoT
* Data rates up to 1 Tbps, 100 times faster than 5G
2. AI-Native Network Architecture Benefits:
* Self-optimizing networks reducing operational costs by up to 50%
* Predictive maintenance decreasing network downtime by 90%
* Dynamic resource allocation improving spectrum efficiency by 40%
3. Intelligent Surfaces and Large Intelligent Surfaces (LIS) Benefits:
* Extends coverage in hard-to-reach areas by up to 25%
* Improves spectral efficiency by 30-40%
* Reduces energy consumption in base stations by up to 20%
4. Ultra-Low Latency (1 microsecond) Benefits:
* Enables real-time remote surgery with haptic feedback
* Supports fully autonomous vehicle communications
* Allows for instantaneous financial transactions and high-frequency trading
5. Quantum Communication Integration Benefits:
* Provides unhackable communication channels
* Enables long-distance quantum key distribution
* Supports ultra-secure government and military communications
6. Enhanced Energy Efficiency Benefits:
* Reduces network power consumption by up to 90% compared to 5G
* Extends IoT device battery life by up to 10 times
* Enables deployment in remote and energy-scarce areas
7. Integrated Sensing and Communication Benefits:
* Centimeter-level positioning accuracy for indoor and outdoor environments
* Supports advanced environmental monitoring and smart city applications
* Enables non-invasive health monitoring through walls
8. Space-Terrestrial Integration Benefits:
* Provides truly global coverage, including remote and oceanic areas
* Enables seamless connectivity for air and maritime transportation
* Supports global IoT deployments with consistent connectivity
9. Brain-Computer Interfaces Benefits:
* Enables direct neural control of devices and systems
* Supports advanced prosthetics with near-natural functionality
* Allows for immersive AR/VR experiences with thought-based interactions
Bottom Line:
The tangible benefits of 6G technology represent a quantum leap in wireless communications capabilities:
1. Speed and Capacity:
100x increase in data rates (up to 1 Tbps) enabling new applications in holographic communication, immersive AR/VR, and massive IoT deployments.
2. Latency:
1000x reduction in latency (to 1 microsecond) unlocking real-time applications in autonomous systems, telesurgery, and financial trading.
3. Energy Efficiency:
90% reduction in power consumption compared to 5G, significantly extending battery life and enabling sustainable, widespread IoT deployments.
4. Coverage:
Near 100% global coverage through space-terrestrial integration, connecting previously unreachable areas and supporting global IoT and transportation systems.
5. Security:
Quantum-safe communications providing unhackable channels for sensitive data transmission and secure government communications.
6. Precision:
Centimeter-level positioning accuracy enabling new applications in indoor navigation, autonomous systems, and environmental monitoring.
7. Intelligence:
AI-native architecture reducing operational costs by 50% and improving network efficiency by 40%, leading to more reliable and adaptive communication systems.
8. Human-Machine Interface:
Direct neural interfaces opening new frontiers in human-computer interaction, healthcare, and immersive experiences.
Quantified Impact:
* Economic Impact:
6G is expected to generate a global economic value of $13.1 trillion by 2035 (based on extrapolated 5G impact estimates).
* IoT Connectivity:
Enabling up to 10 million connected devices per square kilometer, a 10x increase over 5G.
* Industry 4.0:
Potential to increase manufacturing productivity by 30-35% through ultra-reliable low-latency communications and massive machine-type communications.
* Healthcare:
Telesurgery and remote patient monitoring could reduce healthcare costs by 20-25% in developed countries.
* Smart Cities:
6G-enabled smart city solutions could reduce energy consumption by 15-20% and traffic congestion by 30-35% in urban areas.
IBIDG’s Strategic Planning Assumptions and Predictions Report
Strategic Planning Assumptions and Predictions Report
Introduction:
This report compiles predictions and strategic planning assumptions from various reputable sources, including Wall Street Journal, Gartner, IDC, and Forrester. The purpose of this report is to provide a comprehensive overview of anticipated trends and developments in both economic and technological domains. By examining these predictions, organizations can better prepare for potential future scenarios, mitigate risks, and capitalize on emerging opportunities.
The report is divided into two main sections: Economic Predictions and Technology Predictions. Each section contains subsections that focus on specific areas of interest. These predictions serve as valuable inputs for strategic planning processes, helping decision-makers to navigate uncertainty and make informed choices about resource allocation, investment priorities, and long-term strategies.
I. Economic Predictions
Economic predictions provide insights into the future state of financial markets, economic growth, and monetary policies. These forecasts are crucial for businesses and investors to make informed decisions about investments, expansion plans, and risk management strategies.
A. Interest Rates and Monetary Policy
1. With the current federal funds rate at 5.25% as of June 2024, interest rates are likely to decrease by 0.5-1% by June 2025.
2. The prime rate, at 8.5% in June 2024, is expected to adjust by 0.25-0.75% by June 2025, correlating with broader interest rate trends.
B. Housing Market
3. From the current median home price of $428,700 in Q2 2024, the housing market is expected to show a 3-5% increase by Q2 2025, indicating recovery.
C. Economic Growth and Inflation
4. With current GDP growth at 2.1% for Q2 2024, economic conditions will remain highly uncertain, with growth predictions ranging from 1% to 3% for the full year 2024.
5. Assuming current inflation at 3% in mid-2024, significant economic changes are anticipated for the remainder of 2024 and into 2025, with inflation potentially ranging from 2% to 4% by the end of 2024.
D. Stock Market and Investments
6. With the S&P 500 at about 4,500 points in June 2024, the stock market is predicted to experience a shift of 7-10% by June 2025.
7. From its value of approximately $30,000 in June 2024, Bitcoin's price is expected to fluctuate by 20-30% in either direction by December 2024.
E. Global Economic Trends
8. Global economic recovery post-pandemic will be uneven across regions and sectors.
9. Supply chain resilience and diversification will be prioritized.
10. Sustainability and ESG (Environmental, Social, and Governance) factors will increasingly influence business decisions.
II. Technology Predictions
Technology predictions offer insights into emerging trends, innovations, and adoption rates of various technologies. These forecasts help organizations stay ahead of the curve, make strategic technology investments, and prepare for digital transformation initiatives.
A. Artificial Intelligence and Machine Learning
11. By the end of 2024, 80% of large enterprises will have implemented advanced AI-powered cybersecurity solutions, up from an estimated 60% in early 2024. (Gartner)
12. By the end of 2024, 70% of large enterprises will have implemented advanced AI and machine learning solutions in their data analytics processes, up from an estimated 50% in early 2024. (Forrester)
13. AI and machine learning adoption in business processes is forecast to grow by 40% in 2024, with 70% of major companies implementing AI-driven analytics solutions by year-end. (IDC)
B. Cloud Computing
14. Cloud management platform adoption is expected to increase by 25% year-over-year in 2024, with multi-cloud strategies becoming standard for 90% of Fortune 1000 companies. (Gartner)
15. Cloud adoption is expected to increase by 25% year-over-year in 2024, with multi-cloud and hybrid cloud strategies becoming standard for 90% of Fortune 500 companies. (Forrester)
16. Cloud spending is expected to increase by 30% year-over-year in 2024, with multi-cloud strategies becoming standard for 95% of Fortune 1000 companies. (IDC)
C. Internet of Things (IoT) and 5G
17. By Q4 2024, 5G adoption in IoT devices is predicted to reach 50%, up from 30% at the start of the year. (IDC)
18. By Q4 2024, the adoption of emerging technologies in enterprise settings is predicted to reach 50%, up from 30% at the start of the year. (Gartner)
D. Cybersecurity and Data Privacy
19. Increased focus on data privacy will drive 75% of companies to adopt new data protection technologies, increasing their privacy tech budget by at least 20% in the fiscal year 2024-2025. (Gartner)
20. Increased focus on data privacy and security will drive 80% of companies to adopt new data protection technologies, increasing their cybersecurity budget by at least 25% in the fiscal year 2024-2025. (IDC)
21. Increased focus on cybersecurity will drive 75% of companies to adopt new zero-trust security technologies, increasing their cybersecurity budget by at least 20% in the fiscal year 2024-2025. (Forrester)
E. Digital Transformation and Workplace Technologies
22. Human capital management technologies will see continued investment, with 85% of companies expected to use AI-powered HR tools by mid-2025. (Gartner)
23. Digital transformation technologies will see continued investment, with 90% of companies expected to implement advanced digital solutions by mid-2025. (IDC)
24. Digital transformation initiatives will see continued investment, with 85% of companies expected to implement advanced digital workplace solutions by mid-2025. (Forrester)
F. Low-Code/No-Code Platforms and Cloud-Native Development
25. By the end of 2024, 70% of enterprises are expected to be using low-code application platforms for at least a third of their application development. (Gartner)
26. By the end of 2024, 75% of enterprises are expected to be using cloud-native development platforms for at least a third of their application development. (IDC)
27. By the end of 2024, 60% of enterprises are expected to be using low-code or no-code platforms for at least a quarter of their application development and digital innovation initiatives. (Forrester)
G. Edge Computing and Analytics
28. Edge computing adoption is predicted to grow by 50% in 2024, with particular emphasis on IoT and real-time data processing applications. (IDC)
29. Data and analytics capabilities in enterprises are forecast to grow by 35% in 2024, with 60% of major companies implementing advanced analytics solutions by year-end. (Gartner)
H. Customer Experience and Retail Technologies
30. Customer experience technologies are forecast to grow by 30% in 2024, with 65% of major companies implementing AI-driven personalization solutions by year-end. (Forrester)
31. Forrester predicts that retail media networks will grow to an $85 billion market by 2024, with emphasis on personalized advertising and customer data platforms.
Strategic Planning Assumptions:
1. The United States primary economy is entering a new phase of development.
2. Apple will create its own contract-enabled cryptocurrency (probability 0.59).
3. Apple is focusing on AI research and development for healthcare applications across several key sub-markets.
4. There are "Western" eugenics and genetics practices that have shaped society.
5. Inter alias, Persians contributed significantly to Western civilization and culture.
By considering these predictions and assumptions, organizations can better prepare for potential future scenarios, identify emerging opportunities, and develop more robust strategies to navigate the complex and rapidly evolving economic and technological landscape.
Giddeon Gotnor
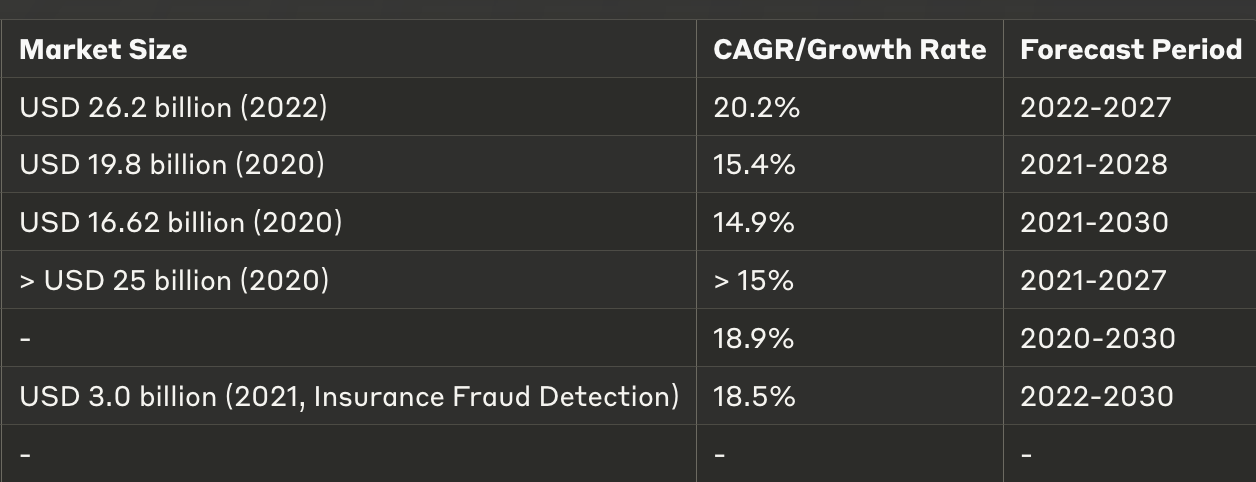
Market Note: Fraud Prevention
Recommended soundtrack: Hypnotize, Biggie Smalls
——
Key Issue: Fraud Prevention
——
Fraud Prevention Industry Report
Introduction
The fraud prevention industry continues to evolve and grow, with a wide range of vendors offering sophisticated solutions to combat increasingly complex fraudulent activities. This updated report provides an overview of the industry, its unique value, key players, their offerings, and the integration of advanced technologies such as biometric authentication and behavioral analytics.
Unique Value of Fraud Prevention
Fraud prevention solutions are critical for businesses to mitigate financial losses, protect their reputation, and maintain customer trust in an increasingly digital world. These solutions leverage cutting-edge technologies, including machine learning, artificial intelligence, big data analytics, and biometric authentication, to detect and prevent fraud in real-time. By proactively identifying and preventing fraudulent activities, businesses can focus on their core operations, ensure regulatory compliance, and provide a secure environment for their customers.
Key Players and Their Offerings
NICE Actimize
Xceed: AI-powered fraud prevention and authentication platform
X-Sight Marketplace: Ecosystem of fraud and financial crime solutions
Feedzai
Feedzai Platform: Real-time fraud detection using machine learning and big data
OpenML Engine: Upgrades fraud prevention models with a single click
DataVisor
User Analytics: Proactive fraud detection using unsupervised machine learning
Forter
Forter Fraud Prevention Platform: Real-time fraud prevention for e-commerce
Kount (now part of Equifax)
Kount Command: AI-driven fraud prevention and digital identity trust
Sift
Sift Digital Trust & Safety Platform: Fraud prevention using machine learning
Ravelin
Ravelin Fraud Detection & Prevention Platform: Real-time fraud detection for online businesses
Simility (now part of PayPal)
Simility Adaptive Fraud Detection: Fraud prevention using machine learning
Signifyd
Signifyd Commerce Protection Platform: Guaranteed fraud protection for e-commerce
BioCatch
BioCatch Behavioral Biometrics: Analyzes user behavior to detect fraud
Integration of Advanced Technologies
Fraud prevention vendors are increasingly incorporating advanced technologies to enhance their solutions' effectiveness:
Behavioral Biometrics
Analyzing user behavior patterns to detect anomalies and potential fraud. Companies like BioCatch and Feedzai are leveraging behavioral biometrics to provide continuous authentication.
Artificial Intelligence and Machine Learning
Utilizing AI and ML algorithms to identify complex fraud patterns, adapt to new threats, and reduce false positives. Vendors such as DataVisor, Forter, and Sift are employing these technologies to improve fraud detection accuracy.
Big Data Analytics
Processing and analyzing vast amounts of data in real-time to identify fraudulent activities. Feedzai and NICE Actimize are leveraging big data to enhance their fraud prevention capabilities.
Ecosystem Approach
Building ecosystems of fraud prevention solutions to provide a comprehensive defense against evolving threats. NICE Actimize's X-Sight Marketplace is an example of this approach.
Bottom Line
Key players in the market are leveraging advanced technologies, such as behavioral biometrics, AI, machine learning, and big data analytics, to provide more effective and efficient fraud prevention solutions. As the digital landscape expands and fraudulent activities become more complex, businesses must adopt a multi-layered approach to fraud prevention, integrating solutions from various vendors to create a robust defense against fraud.
Key Issue: Cool As An Unopened Pack of $2 Bills
Recommended soundtrack: Sympathy for the devil, Rolling Stones
———-
Strategic Planning Assumption: Retirement of the $2 bill will send face value to 10 times the present notional value of the bill. (Probability .69)
Unopened Packs of $2 Bills: A Collector's Guide
Introduction:
Unopened packs of $2 bills are a niche but intriguing area of numismatics. These packs, typically containing 100 sequential bills, offer collectors a chance to own pristine, uncirculated currency directly from the Bureau of Engraving and Printing (BEP).
Sources and Availability:
Federal Reserve:
The primary source of new $2 bills. Banks can order these, but they rarely come in sealed packs for public distribution.
BEP:
Occasionally releases commemorative or special edition packs directly to the public.
Secondary Market:
Auction houses, currency dealers, and online marketplaces sometimes offer sealed packs from various years.
Characteristics:
a) Typically contain 100 bills
b) Bills are in sequential order
c) Wrapped in official BEP packaging
d) Usually include a label with series year and Federal Reserve Bank of origin
Collectibility Factors:
a) Rarity: Truly unopened packs are uncommon, especially for older series.
b) Condition: The sealed nature ensures perfect condition of the bills inside.
c) Series Date: Certain years may be more desirable to collectors.
d) Federal Reserve Bank: Some collectors focus on specific issuing banks.
Value and Investment Potential:
a) Modern unopened packs typically sell for a premium over face value, but not excessively so.
b) Older series or special editions can command higher prices.
c) Long-term investment potential is debatable and depends on various factors.
d) Retirement of the $2 bill will send face value to
10 times to present notional value of the bill. (Probability .69)
Challenges and Considerations:
Authenticity:
Verifying that a pack is genuinely unopened can be difficult.
Storage:
Proper storage is crucial to maintain the pack's integrity.
Temptation to Open:
The sealed nature is key to value, but also limits examination of individual bills.
Legal and Ethical Considerations:
Buying and selling unopened packs is legal.
Some argue that hoarding currency conflicts with its intended circulation purpose.
Collector's Perspective:
For many collectors, the appeal lies in:
a) The pristine condition of the bills
b) The potential for interesting serial numbers
c) The historical aspect of owning a "time capsule" of currency
Market Trends:
a) Interest tends to spike with news about potential discontinuation of $2 bills (though no such plans exist).
b) The market is relatively stable but niche.
Bottom Line
Unopened packs of $2 bills represent a unique intersection of currency collecting and numismatic preservation. While not typically seen as high-value investments, they offer an intriguing option for collectors interested in modern currency in its most pristine form. As with any collectible, thorough research and caution in acquisitions are advised.
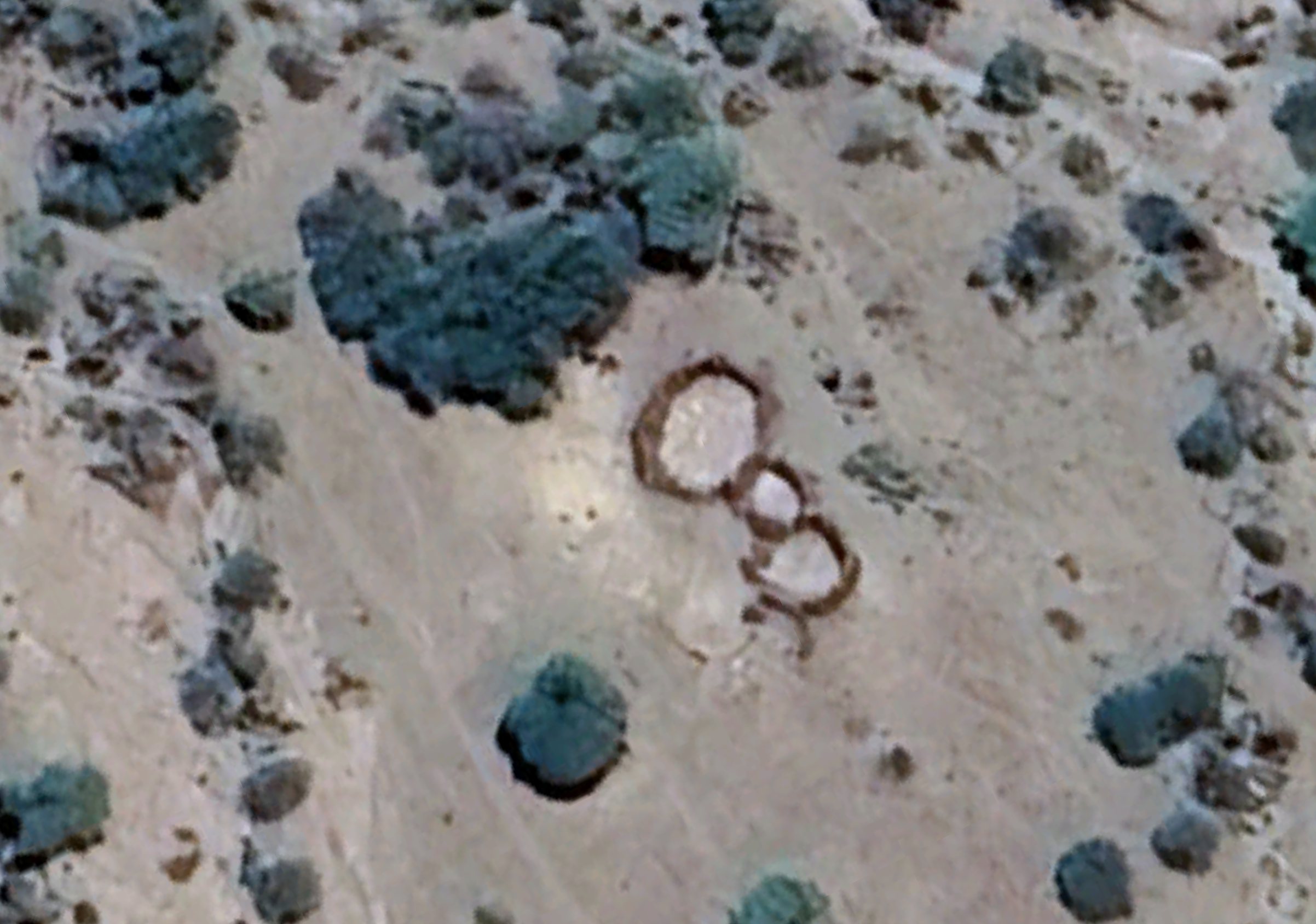
Key Issue: Where Does Artificial Intelligence Estimate The Original “god” Lived ?
god
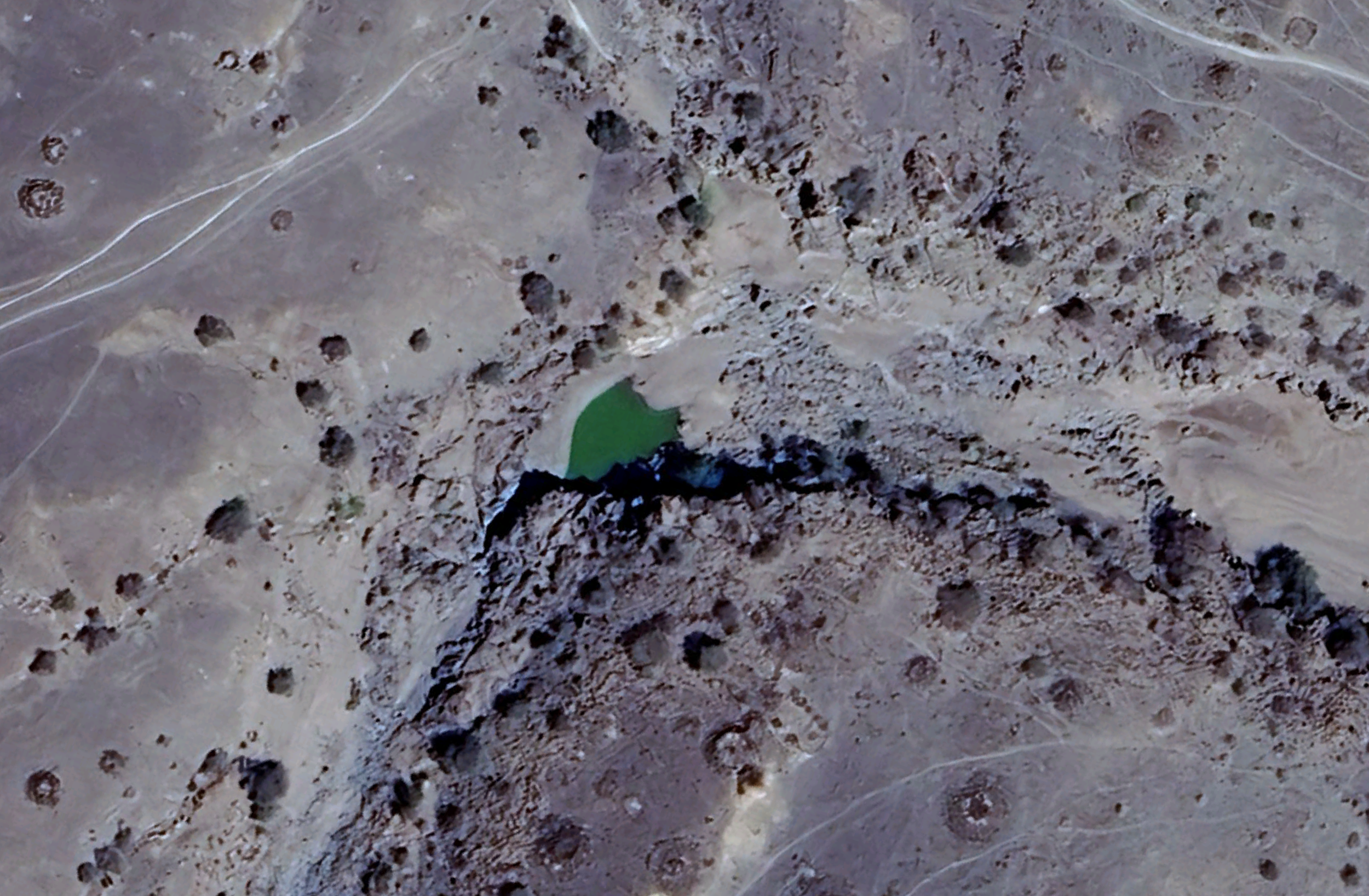
Key Issue: Can Artificial Intelligence Help The World Understand From Where “Sin” Came ?
Key Issue: Can artificial intelligence help the world understand from where sin came ?
—-
Homo Habalis migrated to Russia
Coordinates: 11°20'22.61"N 40°50'18.40"E
Source: East African Rift System, 3.8 million years old site
Source: A primary economic system for Russia
Russia: 55°44'46.76"N 37°38'2.47"E
—-
Ledi-Geraru is a paleoanthropological site located in the Afar Region of Ethiopia. It's significant for several reasons:
Age:
The site dates back to approximately 3.8 million years ago, placing it in the Pliocene epoch. This makes it one of the oldest sites associated with early human ancestors.
Fossil discoveries:
The site is known for fossil remains attributed to early Homo species, specifically Homo habilis and/or Homo rudolfensis. These species are considered some of the earliest members of the genus Homo, marking an important stage in human evolution.
Geographical importance:
Located in the Afar Triangle, this region is part of the East African Rift System, an area rich in paleoanthropological finds. Many crucial discoveries related to human evolution have been made in this general region.
Environmental context:
The fossils found here provide insights into the environmental conditions that early human ancestors lived in, which can help us understand the factors that influenced human evolution.
Nearest city: Semera, the capital city of the Afar Region, is the closest major urban center to the site. This proximity allows for logistical support for ongoing research and excavations.
Ongoing research:
The site continues to be studied by paleoanthropologists and other scientists, contributing to our understanding of early human evolution and the emergence of the genus Homo.
or “an alternative belief system suggests”
1. Sumerian origin:
Many scholars believe the concept of sin originated in ancient Sumerian culture, around 3000 BCE. The Sumerian word "di-im-ma" is sometimes translated as "sin," though its exact meaning is debated.
2. Biblical Hebrew:
In the Hebrew Bible (Old Testament), one of the earliest words translated as "sin" is "חַטָּאת" (chattath), which appears in Genesis 4:7. This verse is part of the Cain and Abel story.
3. Ancient Greek:
In Greek, the word "ἁμαρτία" (hamartia) is often translated as "sin." This term appears in early Greek literature and philosophy, predating its use in the New Testament.
4. Latin:
The Latin word "peccatum" is commonly translated as "sin" and appears in early Christian writings.
5. English:
The Old English word "synn" appears in texts from around the 9th century CE, derived from Proto-Germanic roots.
Evidence suggest: 11°20'22.61"N 40°50'18.40"E East African Rift System, 3.8 million years ago
The East African Rift System (EARS) is a vast geological feature that extends over a significant portion of eastern Africa. Here's a definition of its bounds:
—-
Beatitudo est status mentis qui rationem et cogitationem componit
-
Sign up for IBIDG’s newsletter and you’ll be awesome (Probability .89)