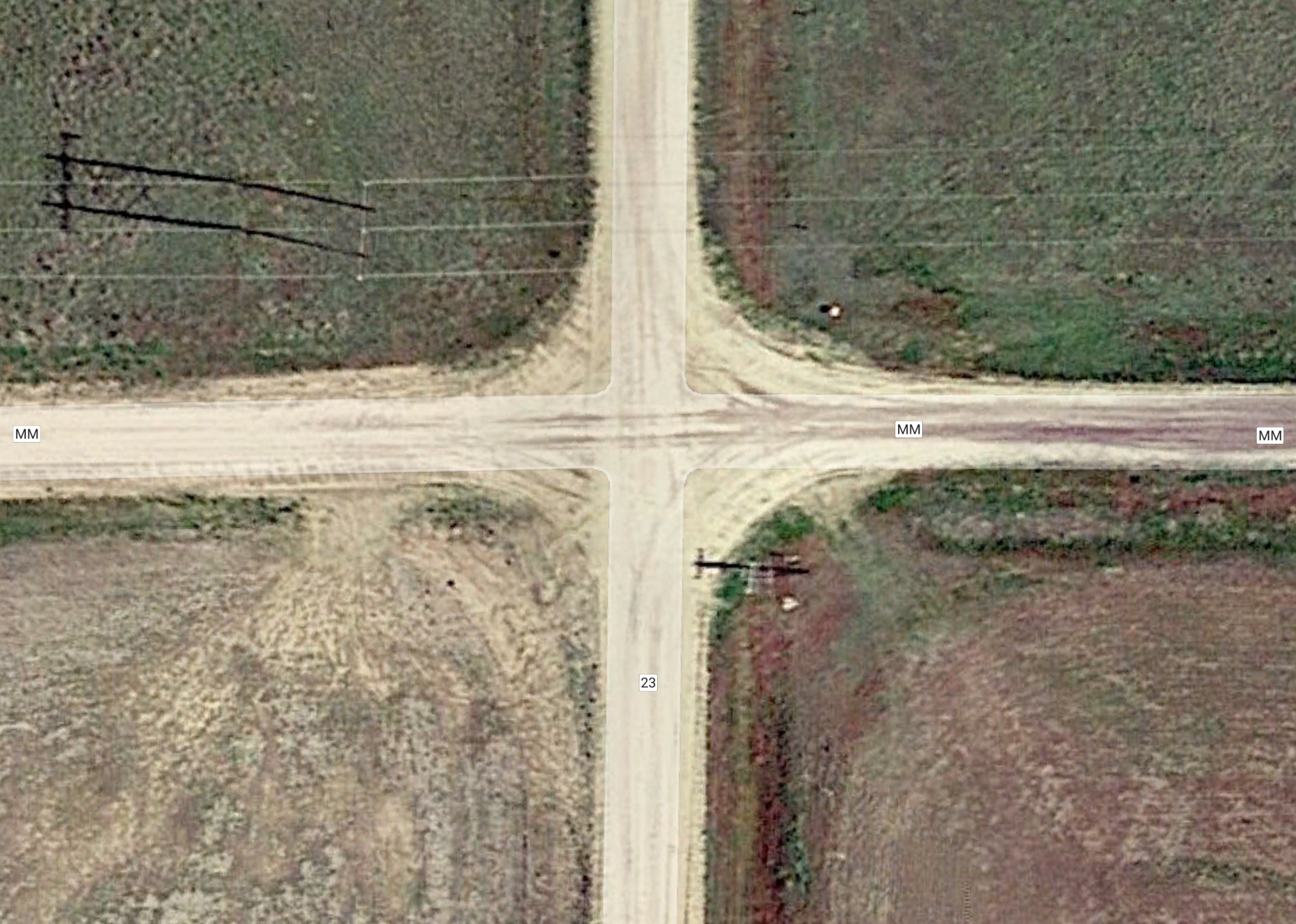
Data Facilities for the Primary Economy
Recommended soundtrack: Hey Man Nice Shot
Key Issue: What Do Under Ground (Primary Economic) Data Facilities Look Like Near Washington D.C. ? (above , a picture of “Cross Roads”)
Data Facilities
1) 38°53'50.34"N 77° 3'51.39"W
2) 38°53'49.86"N 77° 3'49.47"W
IVY League Primary Economic Starting Point
33°46'46.30"N 118°21'35.78"W - Palos Verdes 2/5/43, Wharton
3) Primary Economic System Administration
a) 38°25'48.76"N 77°19'21.52"W
b) 38°25'42.92"N 77°19'20.52"W: 2025 Plan
Defining Three Quantum Hardware Submarkets
A qubit, short for quantum bit, is the fundamental unit of information in quantum computing, analogous to the classical bit in conventional computing. However, unlike classical bits that can only exist in one of two states (0 or 1), qubits can exist in a superposition of both states simultaneously, a phenomenon known as quantum superposition.
Qubits can be realized using various physical systems, but one of the most common implementations is based on superconducting materials. In superconducting quantum processors, qubits are typically made from tiny superconducting loops or Josephson junctions.
Superconducting Loops:
Superconducting loops are small loops of superconducting material, such as aluminum or niobium. In these loops, the circulating current represents the qubit state. The direction of the current flow can be associated with the two states of the qubit: clockwise for state 0 and counterclockwise for state 1. However, due to quantum superposition, the qubit can also exist in a superposition of both states, where the current flows in a combination of both directions.
Josephson Junctions:
A Josephson junction is a thin insulating layer sandwiched between two superconducting materials. This non-linear circuit element can be used as a qubit. The Josephson junction exhibits quantum mechanical effects, such as the Josephson effect, which allows the flow of superconducting current across the insulating barrier. The energy levels of the Josephson junction can be used to represent the qubit states, and by applying microwave pulses, the qubit can be manipulated and controlled.
Working Principle:
In superconducting quantum processors, the qubits are typically cooled to extremely low temperatures, around 20 millikelvin (or even lower), to minimize thermal noise and maintain the quantum properties of the system. At these low temperatures, the materials become superconducting, allowing the flow of current without resistance.
The qubits are manipulated using microwave pulses, which are applied to the superconducting circuits. These pulses can change the qubit state or perform quantum operations, such as single-qubit rotations or multi-qubit entanglement operations. The microwave pulses are tuned to the specific resonant frequencies of the qubits, allowing for precise control over individual qubits or groups of qubits.
Readout of the qubit state is typically performed using a dispersive measurement technique, where a microwave signal is coupled to the qubit, and the state of the qubit modulates the phase or amplitude of the signal. This modulated signal is then amplified and measured, revealing the state of the qubit.
The ability to create and manipulate qubits using superconducting materials and microwave control is a key enabling technology for superconducting quantum processors. These processors are known for their scalability and potential for large-scale integration, as multiple qubits can be fabricated on a single chip using lithographic techniques similar to those used in the semiconductor industry.
1.1 Superconducting Quantum Processors:
Superconducting quantum processors are quantum computing devices that use superconducting circuits to create and manipulate qubits. These processors operate at extremely low temperatures, typically near absolute zero, to take advantage of the quantum mechanical properties of superconducting materials. The qubits in these processors are typically made up of tiny superconducting loops or Josephson junctions, and they are manipulated using microwave pulses. Superconducting quantum processors are known for their scalability and potential for large-scale integration.
Vendors in this submarket include:
1. IBM, 2. Google, 3. Intel, 4. Rigetti Computing, 5. D-Wave Systems, 6. Quantum Circuits Inc. (QCI), 7. Qilimanjaro Quantum Tech, 8. QuTech, 9. Oxford Quantum Circuits, 10. Seeqc, 11. Quantum Microwave, 12. Alice&Bob, 13. Nord Quantique, 14. QuantWare, 15. Qblox, 16. SQC, 17. Anyon Systems, 18. Bleximo, 19. QDevil, 20. Qubit Engineering.
1.2 Ion Trap Quantum Processors:
Ion trap quantum processors use trapped atomic ions as qubits. These ions are held in place using electromagnetic fields, and their quantum states are manipulated using lasers or microwaves. Ion trap quantum processors are known for their long coherence times and high-fidelity operations, making them well-suited for applications that require high precision, such as quantum simulation and quantum sensing.
Vendors in this submarket include:
1. IonQ, 2. Alpine Quantum Technologies (AQT), 3. Honeywell, 4. Universal Quantum, 5. Quantum Brilliance, 6. Oxford Ionics, 7. TMD Technologies, 8. Quantinuum, 9. EeroQ, 10. Qubitekk, 11. AQTION, 12. Trapped Ion Technologies, 13. iXblue, 14. Althea Systems, 15. Aqtion Quantum Technologies, 16. ParityQC, 17. AQT Labs, 18. eleQtron, 19. HQS Quantum Simulations, 20. IonTech.
1.3 Photonic Quantum Processors:
Photonic quantum processors use photons (particles of light) as qubits. These processors manipulate the quantum states of photons using optical components such as waveguides, beam splitters, and phase shifters. Photonic quantum processors are known for their potential for high-speed operation and low power consumption, as well as their compatibility with existing fiber optic communications infrastructure.
Vendors in this submarket include:
1. PsiQuantum, 2. Xanadu, 3. QuiX Quantum, 4. Orca Computing, 5. Quandela, 6. Nu Quantum, 7. Quix, 8. QuTech, 9. Sparrow Quantum, 10. Kets Quantum Security, 11. Qunnect, 12. Quandela SAS, 13. Psi Quantum Computing, 14. QphoX, 15. Qlued Technologies, 16. MPQ Technologies, 17. ORCA Computing, 18. PhotoQ, 19. QuiX Technology, 20. Eagle Quantum Systems.
—————————————————————
In ion trap quantum processors, trapped atomic ions serve as qubits, which are the fundamental units of information in quantum computing. These ions are typically trapped and manipulated using electromagnetic fields generated by electrodes or lasers.
To enable this capability, several natural resources are necessary:
Specific atomic elements:
Different atomic species are used for trapping and manipulating ions, such as beryllium, calcium, strontium, barium, and ytterbium. These elements are derived from various mineral ores found in nature.
Rare-earth elements:
Some ion trap quantum processors utilize rare-earth elements like ytterbium or erbium as the trapped ions. These elements are relatively scarce in the Earth's crust and are often obtained as byproducts from mining other materials.
High-purity materials:
The electrodes, vacuum chambers, and other components used in ion traps require high-purity materials to maintain the required vacuum conditions and minimize interference with the trapped ions. This may involve the use of ultra-pure metals, ceramics, or specialized alloys.
Regarding crystal storage, it does play a role in certain aspects of quantum computing, particularly in the context of photonic quantum processors and quantum communications.
Crystals are important for light communications and storage in the following ways:
Nonlinear optical crystals:
Certain crystals, such as lithium niobate (LiNbO3), potassium titanyl phosphate (KTiOPO4), and beta-barium borate (BBO), exhibit nonlinear optical properties. These crystals are used for efficient generation, manipulation, and detection of single photons, which are essential for photonic quantum computing and quantum communications.
Quantum memories
Crystals like yttrium orthovanadate (YVO4) doped with rare-earth ions (e.g., neodymium, europium, or praseodymium) can be used as quantum memories for storing and retrieving quantum states of single photons. These memories are crucial for synchronizing quantum operations and enabling long-distance quantum communications.
Waveguides and integrated optics
Crystals like lithium niobate and potassium titanyl phosphate can be used to fabricate integrated optical waveguides and circuits for guiding and manipulating photons in a compact and scalable manner, which is essential for photonic quantum computing.

Apple's Chip Research and Development: A Focus on Neural Engine Optimization
Recommended soundtrack: Cowboy, Kidrock
—————————————————————————
Executive Summary: Apple's Neural Engine Chip Research and Development
Apple has been dedicating significant resources to research and development in the field of neural engine chips. An analysis of their development portfolio reveals two primary focus areas: Neural Network Processor Architecture and Optimization (62.1% of R&D time) and Neural Network Task Management and Scheduling (37.9% of R&D time).
Within the Neural Network Processor Architecture and Optimization focus area, Apple is investing in the development of advanced processor architectures that feature multiple neural engine circuits, efficient data handling, scalability, multi-operational modes, and input data processing techniques. These efforts aim to create high-performance, flexible neural engine chips capable of handling complex machine learning tasks.
The Neural Network Task Management and Scheduling focus area showcases Apple's commitment to developing efficient task management systems for neural network processors. This includes the development of neural task manager circuits, priority-based task switching, and techniques for compiling and scheduling transactions, all designed to optimize resource utilization and ensure smooth execution of machine learning tasks on their neural engine chips.
Apple's substantial investment in these two key research and development areas positions the company to develop industry-leading neural engine chips that can tackle the most demanding machine learning applications. As Apple continues to innovate in these areas, we can anticipate the emergence of even more advanced and powerful neural engine chips in the future.

Market Report: A.I. Chips
Recommended soundtrack: Hitchin' a Ride, Vanity Fare
AI Chips Market:
Vendors covered:
1) NVIDIA
2) Intel
3) AMD
4) Qualcomm
5) Apple
6) Google
7) Huawei
8) IBM
9) Samsung
10) Xilinx
11) Arm
12) Alibaba
13) Baidu
14) Graphcore
15) Cerebras Systems
16) SambaNova Systems
17) Mythic
18) Groq
19) Habana Labs (acquired by Intel)
20) Syntiant
21) Horizon Robotics
22) Cambricon
23) Blaize
24) Kneron
25) Hailo
26) Kalray
27) Flex Logix
28) Efinix
29) Tenstorrent
30) Untether AI
AI Chips Market Report
AI Chips Market
$10.14 billion USD in 2020
CAGR of 37.4%
$253.30 billion by 2030,
Market Overview
The AI Chips Market consists of specialized processors designed to accelerate AI workloads, such as deep learning, machine learning, and natural language processing. These chips are optimized to perform complex mathematical computations efficiently, enabling faster and more accurate processing of large datasets. The demand for AI chips has grown significantly in recent years, driven by the increasing adoption of AI technologies across various industries, including healthcare, finance, automotive, and consumer electronics.
Market Size
According to recent market research reports, the global AI Chips Market is expected to grow from USD 10.14 billion in 2020 to USD 253.30 billion by 2030, at a CAGR of 37.4% during the forecast period. This rapid growth is attributed to the increasing demand for AI-enabled devices, the proliferation of cloud-based AI services, and the growing investment in AI research and development by major technology companies.
Market Players
The AI Chips Market is highly competitive, with several key players vying for market share. Some of the major companies in this market include:
NVIDIA:
Known for its powerful GPUs, NVIDIA has established itself as a leader in the AI Chips Market with its Tensor Core architecture and DGX systems.
Intel:
Intel offers a range of AI-optimized processors, including the Xeon Phi and the Nervana Neural Network Processor (NNP) series.
AMD:
AMD's Radeon Instinct accelerators and EPYC processors are designed to tackle demanding AI workloads.
Qualcomm:
Qualcomm's Snapdragon series of mobile processors incorporate AI acceleration capabilities for edge devices.
Apple:
Apple has developed its own AI chip, the Neural Engine, which is integrated into its A-series processors for iPhones and iPads.
Google:
Google's Tensor Processing Units (TPUs) are custom-designed chips for accelerating machine learning tasks in its data centers.
Huawei:
Huawei's Ascend series of AI chips are designed for use in cloud and edge computing scenarios.
Specialization and Collaboration
As the demand for AI chips grows, there is an increasing trend towards specialization and collaboration between technology companies and chip vendors. Many tech giants are partnering with chip manufacturers to develop custom AI processors tailored to their specific needs. This allows them to optimize performance, power efficiency, and cost for their particular AI applications.
For example, Google has partnered with Intel to develop custom TPUs for its data centers, while Apple has collaborated with TSMC to produce its A-series processors with the integrated Neural Engine. These collaborations enable technology companies to differentiate their AI offerings and gain a competitive edge in the market.
Major Areas of Focus
The AI Chips Market is driven by the need to accelerate various AI workloads. Some of the major areas where AI functionality is being programmed into chip sets include:
Computer Vision:
AI chips are being designed to efficiently process and analyze visual data from cameras and sensors, enabling applications such as facial recognition, object detection, and autonomous vehicles.
Natural Language Processing (NLP):
Specialized AI chips are being developed to accelerate NLP tasks, such as language translation, sentiment analysis, and speech recognition.
Recommendation Systems:
AI chips are being optimized to handle the complex algorithms used in recommendation systems, which are commonly used in e-commerce, streaming services, and social media platforms.
Autonomous Systems:
AI chips are crucial components in autonomous systems, such as self-driving cars and drones, where real-time processing of sensor data and decision-making are critical.
Predictive Analytics:
AI chips are being designed to accelerate predictive analytics workloads, enabling faster and more accurate forecasting in various industries, such as finance, healthcare, and supply chain management.
Bottom Line
The AI Chips Market is experiencing rapid growth, driven by the increasing adoption of AI technologies across various industries. As technology companies seek to gain a competitive advantage in the AI space, they are collaborating with chip vendors to develop specialized processors optimized for specific AI workloads. With the continued advancement of AI technologies and the growing demand for faster and more efficient processing, the AI Chips Market is poised for significant growth in the coming years.
Market Report: Analytics and Business Intelligence
Receommended soundtrack: Savage
——————————————————-
Layer 8, Artificial Inelligence Stack
——————————————————
Market Size:
Market size: $23.5 billion
Growth (CAGR): of 8.5%
Definition
The Analytics and Business Intelligence (A&BI) market consists of software applications, tools, and platforms that enable organizations to collect, store, analyze, and derive insights from structured and unstructured data. These solutions help businesses make data-driven decisions, optimize processes, and improve overall performance. According to Gartner, the global A&BI market is expected to reach $23.5 billion by 2025, growing at a compound annual growth rate (CAGR) of 8.5% from 2020 to 2025.
Vendors:
The A&BI market is highly competitive, with numerous vendors offering a wide range of solutions. Some of the key players in the market include:
Microsoft (Power BI)
Tableau (acquired by Salesforce)
Qlik
SAP (SAP Analytics Cloud, SAP BusinessObjects)
IBM (IBM Cognos Analytics)
Oracle (Oracle Analytics Cloud)
SAS (SAS Visual Analytics)
MicroStrategy
Domo
Looker (acquired by Google)
ThoughtSpot
Sisense
Yellowfin
TIBCO Software
Information Builders
———————————————-
Gotnor Group’s Magic Spiral:
Hy-Octane Group:
According to Gotnor Group’s Magic Spiral Microsoft, Tableau, and Qlik are positioned in the Hy-Octane position. These vendors demonstrate strong execution capabilities and a clear vision for the future of A&BI. They offer comprehensive and mature solutions that cater to a wide range of use cases and industries.
Chi Guys
SAP, Oracle, and IBM are positioned in the Challengers spiral. These vendors have a strong presence in the market and offer robust A&BI solutions. However, they may lag behind the Leaders in terms of innovation or may have a more limited focus in terms of target markets or industries.
Vendors That-Go
SAS, MicroStrategy, and ThoughtSpot are positioned in the “Vendors that-go” or Visionaries. These vendors demonstrate a strong understanding of emerging trends and offer innovative capabilities in areas such as augmented analytics, natural language processing, and AI-driven insights. However, they may have a more limited market presence compared to the Hy-Octane Group and Challengers.
Sleigh Riders:
Domo, Yellowfin, and TIBCO Software are positioned in the Sleigh Riders position on the spiral. These vendors offer specialized A&BI solutions that cater to specific use cases, industries, or market segments. While they may not have the broad market presence of the Hy-Octane Group and Challengers, they can be a good fit for organizations with specific requirements.
Bottom Line:
The Analytics and Business Intelligence (A&BI) market is a dynamic and growing space, with vendors constantly innovating to meet the evolving needs of organizations seeking to harness the power of data-driven insights. Gotnor Group’s Magic Spiral provides a valuable framework for evaluating the strengths and weaknesses of A&BI platforms, helping businesses make informed decisions when selecting a solution.
Microsoft, Tableau, and Qlik lead the pack, offering comprehensive and mature A&BI platforms that cater to a wide range of use cases. SAP, Oracle, and IBM are strong challengers, while SAS, MicroStrategy, and ThoughtSpot are visionaries driving innovation in emerging areas. Niche players like Domo, Yellowfin, and TIBCO Software offer specialized solutions for specific requirements.
As the market continues to evolve, organizations should carefully evaluate their A&BI requirements, considering factors such as ease of use, scalability, AI capabilities, and integration with existing systems. By selecting the right A&BI platform, businesses can unlock the full potential of their data and drive better decision-making across the enterprise.
Coverage: Technology Markets
IBIDG Market Coverage
1) Power and Resources
2) AI Chipsets and Accelerators: Hardware components designed to accelerate AI workloads, including GPUs, ASICs, and FPGAs. (Layer 3)
3) AI Frameworks and Libraries: Software tools and libraries that facilitate the development and deployment of AI models, such as TensorFlow, PyTorch, and scikit-learn.
4) (Layer 4) AI Algorithms and Models: Various AI algorithms and model architectures used for tasks such as machine learning, deep learning, natural language processing, and computer vision.
5) AI Training Datasets: Diverse and high-quality datasets used to train
6) AI models across different domains and applications.
7) AI Safety and Ethics: Practices, guidelines, and tools to ensure the development and deployment of safe, ethical, and trustworthy AI systems.
8) AI Applications and Platforms: End-to-end AI solutions and platforms that enable organizations to build, deploy, and manage AI applications across various industries and use cases.
9) Analytics and Business Intelligence (Layer 8)
10) API Management (Layer 8)
11) Application Performance Monitoring (APM) (Layer 8)
12) Backup and Recovery (Layer 8)
13) Business Process Management (BPM) (Layer 8)
14) Cloud Infrastructure as a Service (IaaS) (Layer 8)
15) Contact Center as a Service (CCaaS) (Layer 8)
16) Content Collaboration Platforms (Layer 8)
17) Content Services Platforms (Layer 8)
18 ) CRM Customer Engagement Center (Layer 8)
19) CRM Lead Management (Layer 8)
20) Data Center Backup and Recovery (Layer 8)
21) Data Center Networking (Layer 8)
22) Data Integration (Layer 8)
23) Data Loss Prevention (DLP) (Layer 8)
24) Data Quality (Layer 8)
25) Data Science Platforms (Layer 8)
26) Data Warehouse and Data Management Solutions (Layer 8)
27) Digital Commerce (Layer 8)
28) Digital Marketing Analytics (Layer 8)
29) Digital Marketing Hubs (Layer 8)
30) Distributed File Systems and Object Storage (Layer 8)
31) Enterprise Architecture Tools (Layer 8)
32) Enterprise Content Management (ECM) (Layer 8)
33) Enterprise Information Archiving (Layer 8)
34) Enterprise Network Firewalls (Layer 8)
35) Enterprise Risk Management (Layer 8)
36) Field Service Management (Layer 8)
37) Financial Corporate Performance Management (Layer 8)
38) Full Life Cycle API Management (Layer 8)
39) Horizontal Portals (Layer 8)
40) Hyperconverged Infrastructure (Layer 8)
41) Identity Governance and Administration (Layer 8)
42) Industrial IoT Platforms (Layer 8)
43) Integrated IT Portfolio Analysis Applications (Layer 8)
44) Integrated Revenue and Customer Management for CSPs (Layer 8)
45) Integrated Risk Management (Layer 8)
46) Integrated Workplace Management Systems (Layer 8)
47) IT Service Management Tools (Layer 8)
48) IT Vendor Risk Management (Layer 8)
49) Managed M2M Services (Layer 8)
50) Managed Security Services (Layer 8)
51) Manufacturing Execution Systems (Layer 8)
52) Master Data Management (Layer 8)
53) Metadata Management Solutions (Layer 8)
54) Mobile Device Management (Layer 8)
55) Modular Servers (Layer 8)
56) Multichannel Campaign Management (Layer 8)
57) Multichannel Marketing Hubs (Layer 8)
58) Network Firewalls (Layer 8)
59) Network Performance Monitoring and Diagnostics (Layer 8)
60) Network Security (Layer 8)
61) Procure-to-Pay Suites (Layer 8)
62) Public Cloud Infrastructure as a Service (Layer 8)
63) Sales Force Automation (Layer 8)
64) Secure Web Gateways (Layer 8)
65) Security Awareness Computer-Based Training (Layer 8)
66) Security Information and Event Management (SIEM) (Layer 8)
67) Unified Communications as a Service (UCaaS) (Layer 8)
68) Unified Endpoint Management (Layer 8)
69) Unified Threat Management (Layer 8)
70) User Authentication (Layer 8)
71) Utilities Customer Information Systems (Layer 8)
72) Warehouse Management Systems (Layer 8)
73) Web Application Firewalls (Layer 8)
74) Web Content Management (Layer 8)
75) Wired and Wireless LAN Access Infrastructure (Layer 8)
76) Workforce Analytics (Layer 8)
77) Workforce Engagement Management (Layer 8)
78) Application Security (Layer 8)
79) Endpoint Security (Layer 8)
80) Cloud Security (Layer 8)
81) Security Services (Layer 8)
82) Mobile Security (Layer 8)
83) Artificial Intelligence (AI) in Healthcare (Layer 8)
84) Cybersecurity (Layer 8)
85) AI Distribution & Ecosystem (Layer 9)
86) Human & AI Interaction (Layer 10)
87) Monetization (Layer 11)
Note: Markets related to specific AI applications in healthcare have been categorized as spanning both Layer 8 (AI Application & Integration) and Layer 11 (Monetization). The majority of the other markets fall under Layer 8, as they represent various software applications, platforms, and services that integrate AI capabilities.
Beatitudo est status mentis qui rationem et cogitationem componit
-
Sign up for IBIDG’s newsletter and you’ll be awesome (Probability .89)